Are your knowledge sharing strategies lacking?
Something curious is happening with respect to knowledge management. One look around would suggest that, before the pandemic and so-called Great Resignation, knowledge management was dead. A dusty backoffice relic of enterprise operations now revived by the needs of distributed workforces.
Article after internet article positions knowledge management and, by extension, knowledge sharing, as critical all of a sudden, as opposed to what it has always been: critically overlooked. Way back in 2020, a survey from Deloitte found that three quarters of companies agreed on the importance of knowledge sharing, while only 9% were prepared to enact knowledge-sharing processes.
Sure, the global pandemic and dispersed workforces put knowledge management strategy back under the microscope. But the central issue remains the same: employees, customers, and partners can’t find what they need as easily as they’re used to in other channels.
It’s what we call the “wow” gap.
Wait, What’s a “Wow” Moment?
A wow moment is one that helps people do more on their own with less effort. It’s enabled by knowledge sharing—that is, providing relevant, personalized, and contextual knowledge right in the moment of need.
Wow moments go beyond knowledge management and content strategy or delivering search results. They account for context. They capture and act on user intent. And they provide real-time recommendations through the power of AI. It’s no wonder that wow moments have tremendous power to alleviate customer frustration and poor experiences.
Welcome to the Wow Gap
As much as we crave and consume it, we don’t always share organizational knowledge and expertise. Nor do we integrate collective knowledge into our customer strategies. This loss of company knowledge, which is more prevalent within organizations, is causing a wow gap on the customer journey.
The wow gap is the great divide between the personalized, relevant experiences that customers get from other service providers, versus their experience with your company. Because let’s face it: Google and Amazon make it incredibly easy to find things. Their institutional knowledge sharing strategies are superb. Once you grow accustomed to that caliber of experience, anything less will stand out like a sore thumb.
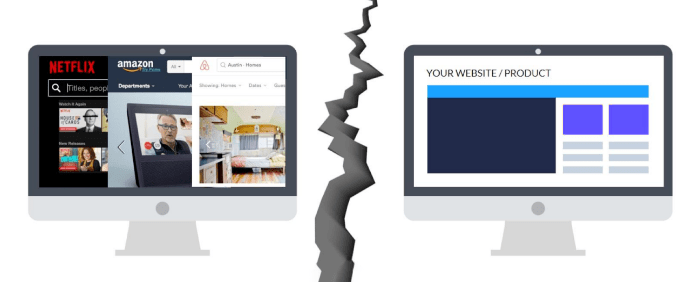
Try as enterprises might to create knowledge sharing culture and wow their people, scalability remains a problem—especially as businesses scale and workplaces evolve to a more distributed global model. It’s impossible to manually track, manage, and analyze. Not if you want to wow.
Here’s our simple model for creating wow moments at scale:
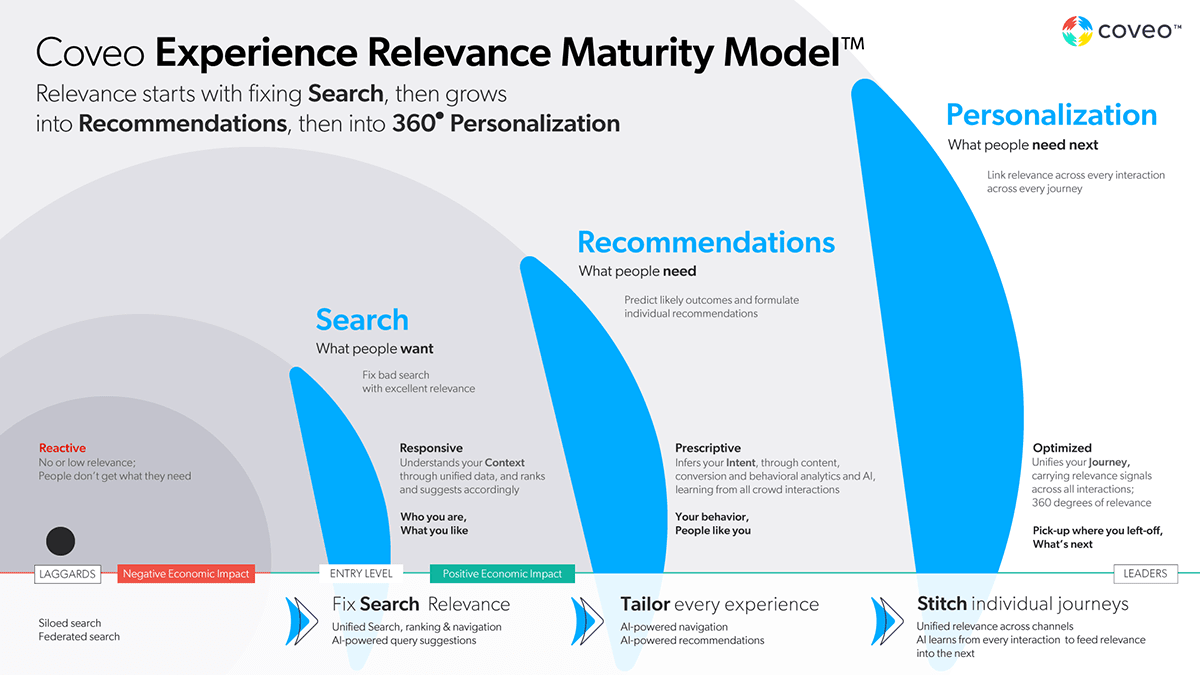
Seems simple enough, right? Content (your knowledge ecosystem) + context (your apps, portals, consoles, and devices) + behavior (your predictive analytics) = relevance (powered by your ML recommendations).
So why are 34% of employees still frustrated with “chaotic, disorganized” information repositories? And why are three of APQC’s top 5 KM priorities related to knowledge sharing, namely:
- Transferring expert knowledge (24%)
- Enabling collaboration across teams/units (24%)
- Improving content management practices (23%)
Probably because strong knowledge sharing supports a variety of organizational objectives. We see the value reflected in improved internal collaboration and communication.
Typically, organizations with effective knowledge sharing onboard agents faster while deflecting more “tier 1” tickets. Agents get what they need in the moment and, ideally, customers get what they need long before an agent interaction becomes necessary. As to what knowledge transfer looks like in practice, here are seven avenues for creating wow moments across customer and employee journeys.
7 Knowledge Sharing Strategies to WOW Your Customers
1. Elevate Self-Service Search
Make it easy for your people to help themselves. Unify knowledge from a variety of sources and infuse customer communities and self-service portals with relevant resources. This could be a quick knowledge base article that solves a particular issue.
Let’s be real, though: a lot of self-service journeys begin with Google—before a person reaches a company’s website. Think about allowing Google to index your self-service content so customers can get what they need where they go for answers first.
2. Make Better Recommendations
Through rich usage analytics, you can learn what helped other customers with a similar query and successfully predict what they’ll need next. Intelligent recommendations enhance the customer experience, build brand loyalty, and drive more conversions.
According to the aforementioned APQC report, 44% of knowledge management professionals think “AI to recommend content or knowledge assets” will be the most important KM tech over the next three years.
Indeed, machine learning helps to track which resources have proven to be the most helpful and gives deeper insight into what your customers are looking for and what content gaps you may have.
3. Deflect Cases So Customers Don’t Have to Call
You’re likely familiar with a common form of case deflection: the case submission form. As customers type in their issue, they’re shown potential solutions (that would make the case submission unnecessary).
Case deflection isn’t a new customer service concept. But some enterprises are finding savvy ways to enhance case deflection through advanced knowledge sharing.
For example, insight engines use a combination of machine learning and usage analytics to effectively measure and improve case deflection. Enterprises can use this information to continually improve their self-service experiences, closing the loop on top issues and supporting wow-worthy case deflection.
4. Extent Content to In-App Experiences
AI-powered search can extend beyond your website, communities, and portals. Innovative companies are helping their customers find the knowledge they’re looking for by offering contextual recommendations directly within the software and services they offer.
5. Enable Agents to Work Smarter
Empowering your agents with the most relevant knowledge directly within their case console will not only increase their proficiency, it will reduce attrition and protect your brand. The idea is to limit context switching and equip agents with real-time recommendations based on the customer’s profile, case information, and activity prior to submitting the case.
Providing knowledge sharing tools to agents tends to ease agent burnout and improve average handle time, especially for common cases that other agents have already found solutions for.
6. Translate and Localize Support Content
This is one of the more overlooked aspects of successful knowledge management. Most enterprises have customers and employees in different countries or regions. Multi-national or multi-regional enterprises can extend low-friction experiences to more of their audience just by translating and localizing their existing knowledge.
Coveo, for example, allows you to change the language of your search interface.
7. Capture Employee Knowledge
While it’s important to make sure you don’t lose valuable knowledge when you lose employees, it’s just as important to enable employees to capture, share, and improve the knowledge they already have. This is a guiding principle of knowledge-centered service.
According to KCS Academy, a strong culture of knowledge capture and transfer can improve employee retention by 20-35% (among many other benefits). Consistent knowledge creation is critical to both employee and customer success.
Sharing is Caring! Unify and Extend Shared Knowledge for More Wow Moments
I don’t know about you, but I feel wowed when enterprises anticipate my needs—when it feels like they already know me and what I need to get on with my day. That could be a personalized content recommendation within an app I’m using, or an especially well-prepared support agent who quickly solves my issue.
At the core of these low-friction experiences is effective knowledge sharing. The enterprises who not only create a culture of knowledge sharing, but undergird that culture with the right technology and processes, will find their wow gaps fewer and far between.