Jump started by the uber popularity of ChatGPT, a large language model (LLM) developed by OpenAI, artificial intelligence is entering a new phase. It’s changing the way we communicate, create, and work daily. The excitement is centered around the generative ability of computers, or generative AI – a subset of machine learning. Generative AI models can be used to create new ideas, content, and 3D models from natural language prompts and training data.
As of November 2023, 23% of global CEOs and 32% of global CMOs surveyed by Statista said they’d already adopted AI into their operations with 43% and 39% of CEOs and CMOs, respectively, saying they plan to explore options for adoption in the future. The most popular AI use cases included service operations optimization at the top. It was followed by the creation of new AI-based products and solutions. With ChatGPT taking generative AI mainstream throughout 2023, the development and adoption of new generative AI tools to augment human capabilities will only increase.
What you need to know to take advantage of generative AI for your business in this rapidly evolving environment? Let’s unpack.
What Is Generative AI?
Generative AI is a type of artificial intelligence that creates something new and original from existing data using a machine learning model. It represents a significant advancement in AI capabilities. GenAI uses deep learning models that can learn from data automatically without being programmed to do so.
LLMs, such as OpenAI’s GPT series (Generative Pre-trained Transformer) and the conversational AI application ChatGPT, are a type of generative AI specifically designed for natural language generation. These models are trained on massive volumes of data and use deep learning to generate human-like text. The latest models are impressive for their range of abilities from drafting emails to generating code.
In addition to text, today’s generative AI solutions can create new images, music, simulations or computer code. DALL-E, also from OpenAI, is an AI tool that generates entirely new and realistic images from natural language descriptions using a combination of natural language processing and computer vision techniques.
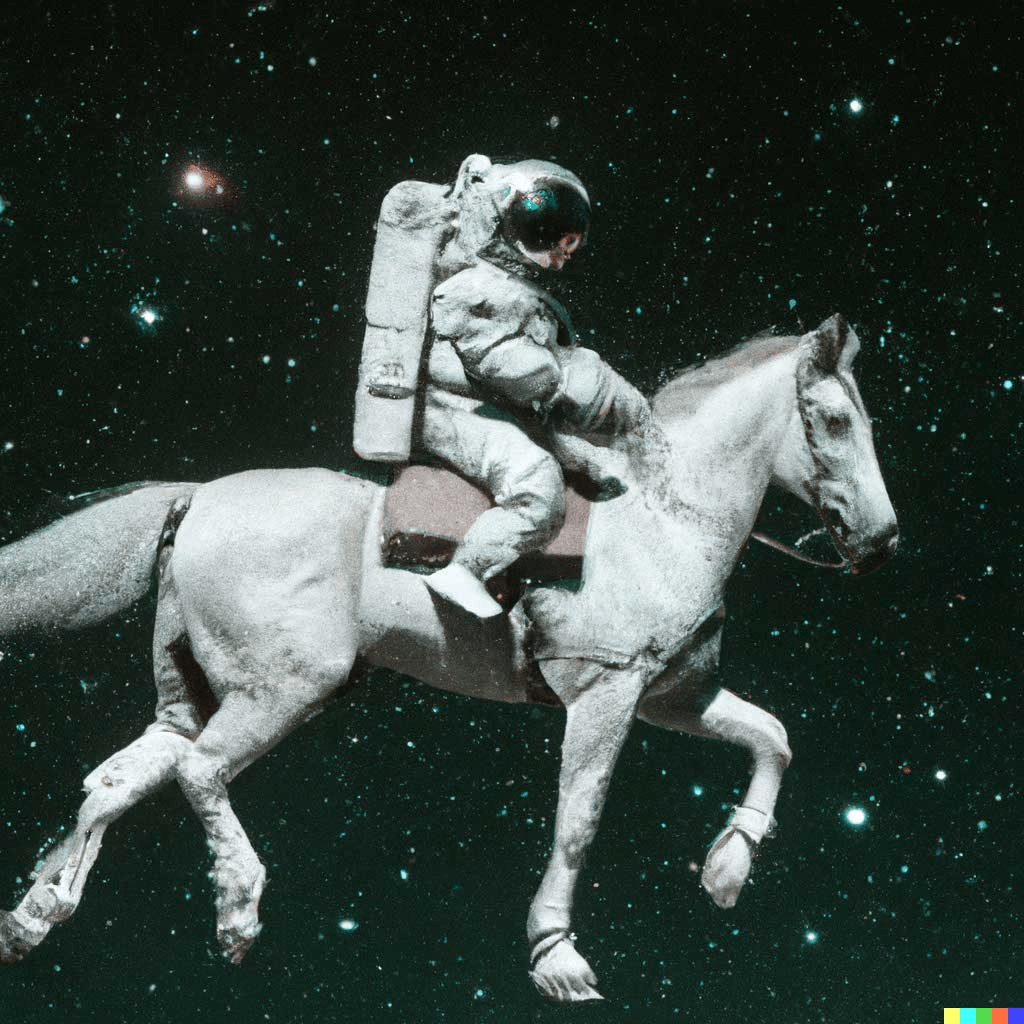
How Does Generative AI Work?
Generative AI works by using machine learning algorithms, specifically deep learning neural networks, to detect patterns and relationships in training data. It uses this information to generate entirely new synthetic data or content. The starting point to generative AI is the collection of large amounts of data from various sources that contain content such as text, images, audio, or code. This data is then preprocessed and fed into a generative AI model that learns patterns, relationships, and nuances in the data through a process called training.
The model learns a probability distribution across its training data and uses that distribution to generate new data similar to the original. A key point here is that the new content is unique. The model creates similar content without simply copying or and reproducing the training data. It uses an iterative process of adjusting the foundation model’s parameters until it can generate convincing novel outputs.
A deep learning neural network allows an AI model to process large amounts of data, using complex algorithms to identify patterns and continuously learn. A neural network is made up of layers of interconnected nodes designed to mimic the structure of the human brain. Over time, the AI model improves its performance by comparing its generated output against the expected output and making adjustments, using techniques like backpropagation to update the strength of connections between nodes.
For example, a large language model like GPT-3 uses a massive neural network to analyze text data, such as Wikipedia articles and novels, to make predictions based on that data and produce new text when prompted. In cases like ChatGPT, the AI model can carry conversations and develop new skills such as essay writing, translations to different languages, and computer coding.
Once a generative model has been developed and trained, humans can fine tune the generative AI system for specific tasks and industries by feeding it additional data, such as for biomedical research or satellite imagery in the defense industry.
What Can Generative AI Do?
The uses of generative AI are far reaching, touching all industries including robotics, music, travel, medicine, agriculture, and more. Its potential applications are still being explored and developed. Generative AI’s major appeal is in its ability to produce high-quality new content with minimal human input. Companies outside of tech are already taking advantage of generative AI tools for their ability to create content so good it sometimes seems human made. For example, clothing brand Levi’s announced they will use AI-generated virtual models for clothing image generation to provide its customers with more diverse shopping experiences.
When it comes to language, all industries or job functions that depend on clear and credible writing stand to benefit from having a close collaborator in generative AI. In the business world, these can include marketing teams that work with generative AI technology to create content such as blogs and social media posts more quickly. Tech companies can benefit from coding generated by AI, saving time and resources for IT professionals so they can pursue opportunities for creating greater value for their businesses.
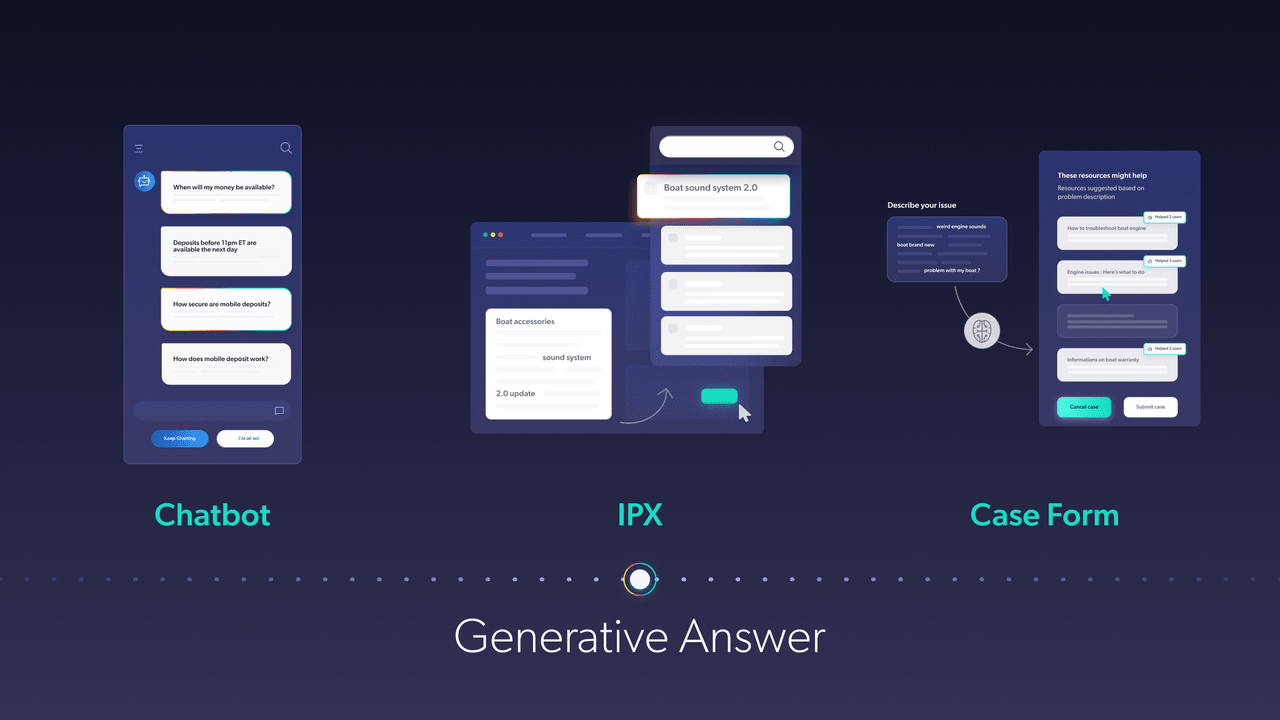
The customer service industry, in particular, is poised for incredible gains from this technology. By 2026, conversational AI will cut the labor costs of contact centers by $80 billion, according to Gartner. Conversational AI will make agents more efficient and effective with one in 10 agent interactions set to be automated by 2026. AI models will allow support agents to dedicate themselves to different and better work.
Let’s take a closer look at what generative AI can do for businesses.
For the Contact Center
Generative AI has the potential to significantly benefit the contact center for both agents and customers. Currently many organizations struggle with agent staff shortages and high labor expenses, which make up 95% of contact center costs, according to Gartner. Companies who use generative AI to automate even parts of interactions, such as identifying a customer’s policy number, will greatly reduce time spent on tasks typically supported by humans.
AI-powered chatbots can use natural language processing to perform a sentiment analysis, empathize with unhappy customers and improve their experiences. Using both unsupervised and supervised learning, advanced language models can answer questions and have conversations with customers across both text and voice channels.
For Knowledge Base and Search
People working in customer service are faced with the need to learn and access a lot of information to serve customers. There is a paradigm shift happening in support organizations from search to answers. Generative AI can improve the self-serve knowledge base experience to help customers find answers quickly and intuitively on their own.
With LLMs, customers will be able to ask a question and get a high quality answer right away, structured like a conversation with a human, instead of the traditional list of results. To accomplish this, companies will need to invest in training machine learning using techniques like supervised learning to fine-tune them to their specific domains.
We will cover generative AI’s impact on search further below.
For Personalization
Generative AI can be a powerful way to create interactions with customers at a deeply personal level. AI models are capable of analyzing massive amounts of customer data, such as purchasing behavior and profile data, to understand what a customer wants and respond in a human-like way. With continual learning, the AI model will improve its performance on serving customers as it gathers more information and learns through trial and error over time.
In the area of customer self service, generative AI can also help solve the comprehension problem. This is the failure of getting a customer a satisfactory answer because the content isn’t relevant to them or they don’t understand the terminology. AI can fill in the gaps that lead to failures in addressing customers’ inquiries by automatically adjusting content to their levels of expertise or experience.
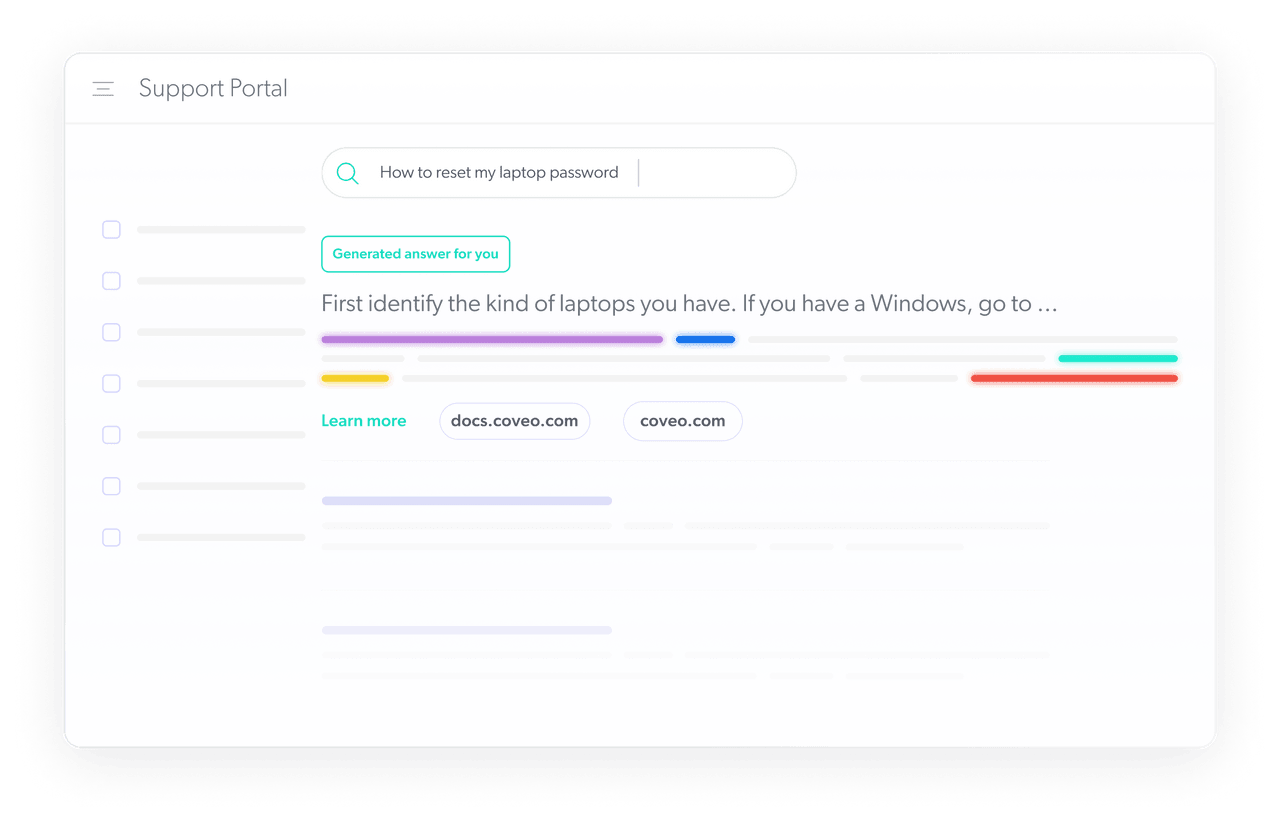
Content Creation
Enterprise content is key to shifting the cost structure from labor involved in delivering answers to developing LLMs to perform the heavy lifting. Agents and knowledge workers can lean on generative AI to draft content in their areas of expertise to produce high-quality content for the knowledge base, product documentation and help articles. This in turn builds up the repository of content necessary for LLMs to assemble the best answers for customers.
Beyond text, generative AI models like diffusion models can create realistic images and generate synthetic data, enabling new possibilities in content creation. Ecommerce companies can use LLMs and image generation models to create content for product catalogs — including product images, descriptions, and specifications, and help create instruction manuals and other sales collateral.
What Are AI Hallucinations?
As impressive as generative AI is, there are risks involved in using these powerful tools. The outputs from these AI models seem accurate and convincing, but it’s become clear that they often repeat wrong information. For example, several people have recorded instances when ChatGPT referenced studies that did not actually exist. This tendency for AI models to produce something that appears completely true but is made up has been coined “hallucinations.”
Hallucinations pose a significant challenge for the responsible development and deployment of generative AI applications. As businesses increasingly adopt these technologies, it is crucial to address this issue to ensure the reliability and trustworthiness of AI-generated content.
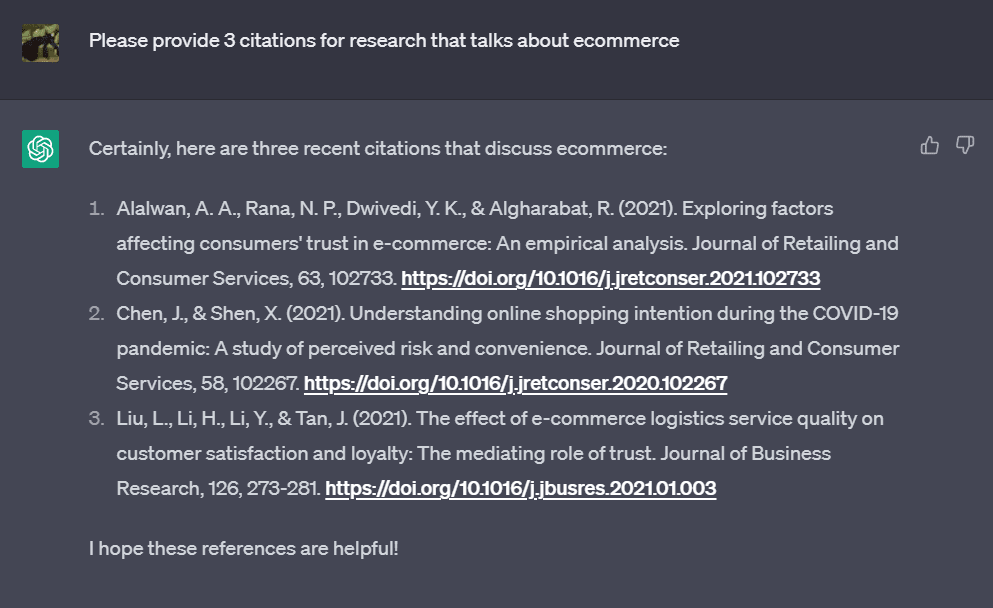
Why do hallucinations occur? One reason could be that the AI model makes deductions from incorrect, inadequate, insufficient and or outdated training data used during the development of the AI system. There is no shortage of “fake news” on the web, and unfortunately it can get regenerated as fact. Recognizing factual information versus misleading or false data is a complex task that requires advanced data science techniques and rigorous quality control measures.
In some cases these hallucinations are easy to spot and amusing. But other times, they can have serious consequences. For businesses, misinformation and inaccuracies can lead to an erosion in customer trust and harm to their reputations. Generating false or misleading information has ethical – and often legal – implications, thus there’s a strong need to implement responsible AI practices.
While generative AI such as ChatGPT can be a great benefit to businesses, organizations will want to put safeguards in place to account for AI models’ inabilities to ensure accuracy or to discern truth. One way to mitigate hallucinations is to fine-tune pretrained models to enterprise-specific data so they are not relying on their internal knowledge.
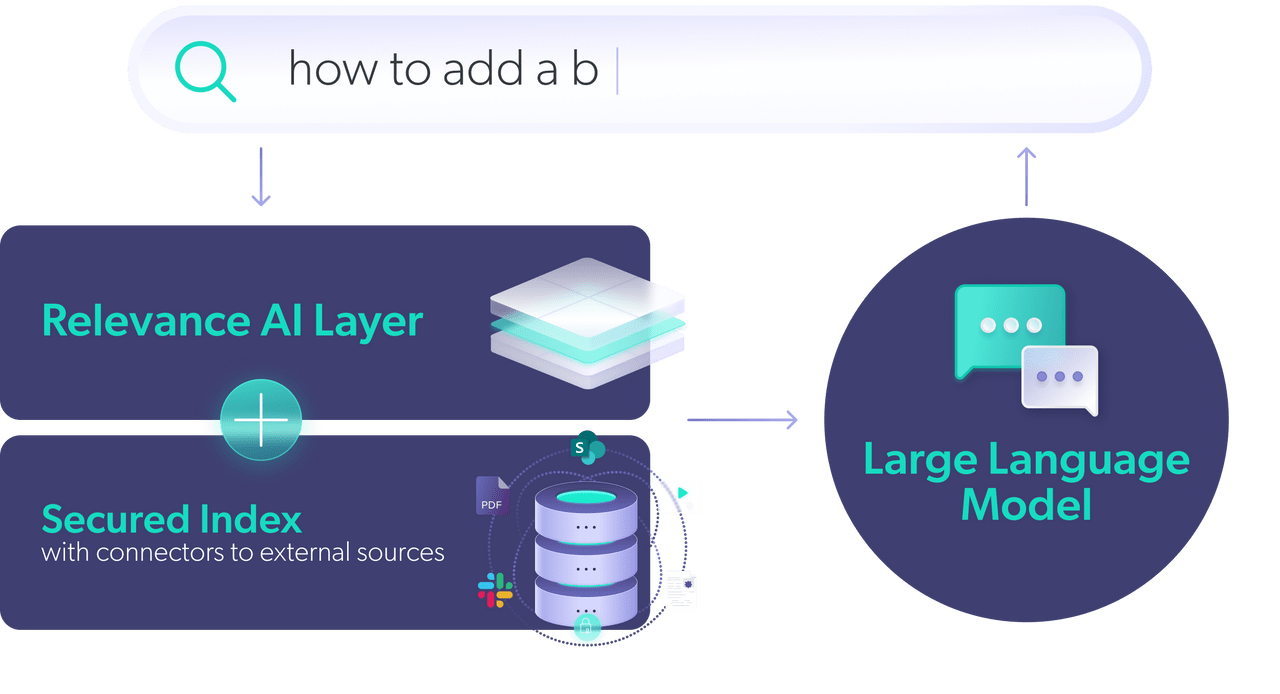
For example, a system like Coveo surfaces using retrieval augmented generation that pulls results first first from a unified index and then uses an LLM to construct an answer, with links to original sources, can minimize hallucinations and make fact checking possible. Additionally, a layer of human supervision is necessary to verify the factual accuracy of AI, especially in scenarios where accuracy is paramount.
Another approach to reducing hallucinations involves using advanced AI architectures like generative adversarial networks (GANs). In a GAN, two neural networks, a generator and a discriminator, are trained simultaneously. The generator creates new data instances while the discriminator evaluates them for authenticity. This adversarial process can help improve the quality and reliability of the generated output.
Technology isn’t the only safeguard you can implement to prevent hallucinations. Creating a robust AI governance strategy ensures the responsible development and use of generative AI applications. This includes establishing clear guidelines, protocols, and oversight mechanisms to monitor and validate the outputs of AI models. A comprehensive AI strategy should also prioritize transparency, accountability, and the ability to interpret and explain the decisions made by AI systems.
And as a final safeguard, you can limit the AI system’s access to sensitive information to prevent unintended disclosures of confidential information and account for the unpredictable nature of language models’ responses.
Will Generative AI Replace Search?
That brings us to the question of whether generative AI will take over for search. We’ve covered the tendency of generative AI to hallucinate answers, which would impact the trustworthiness of search results, but there are other risks and limitations involved in deploying these AI models in real-world applications..
The large language models that get trained on massive amounts of texts on the internet are also reflecting the biases that may be present in its source materials. This poses a danger for companies in producing responses to customers that are discriminatory, highlighting the need for responsible AI practices and robust data science techniques to mitigate biases.
Search relies on the most up-to-date data, but LLMs work from fixed knowledge and it would take an incredible amount of resources and expenses to retrain them continuously to ensure data freshness.LLMs can be vulnerable to data extraction attacks, putting sensitive and confidential information at risk. Ensuring data security and privacy is a critical component of any AI strategy involving generative models.
Due to these limitations and risks, generative AI isn’t a replacement for search. But generative AI can significantly augment search by providing more natural, conversational, and context-aware interactions with users. After all, there are many instances when all we want from a search is “the answer” or a summary of the main points in an understandable manner, both scenarios that we can get closer to achieving with generative AI applications.
At Coveo, our approach is to use generative AI in conjunction with search to create a digital experience that is trustworthy, secure and up to date in its results. We recommend using large language models to assemble the answer and rely on a company’s enterprise content for the data. This can be achieved through Retrieval-Augmented Generation (RAG), which is a promising technique that combines the strengths of retrieval-based and generative approaches. RAG involves finding relevant documents first and then using LLMs to generate the answer.
A secure search technology used with generative AI can also ensure the permissions of source systems are always in place and you are respecting the privacy of your customers.
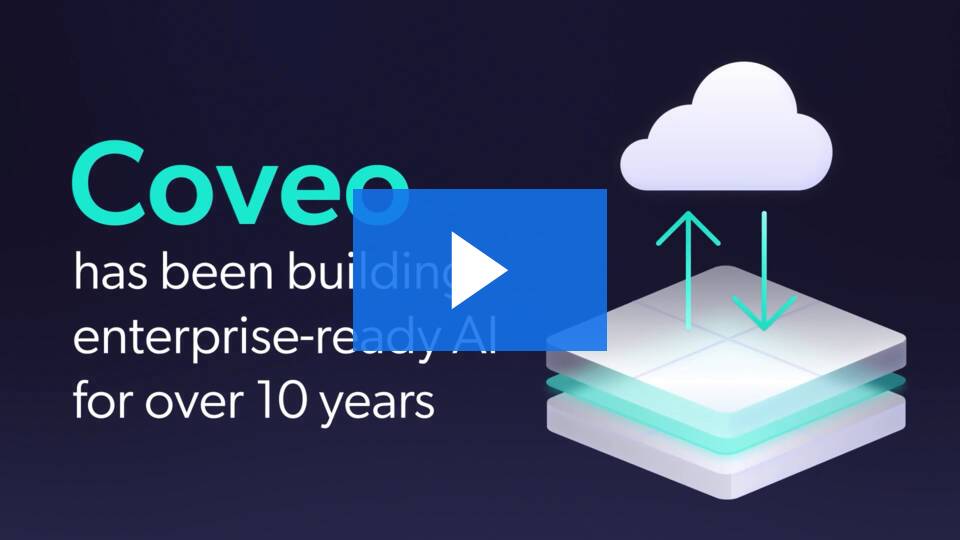
Coveo Relevance Generative Answering: Join the Beta Today
Ending Thoughts
Generative AI is a relatively new field that is quickly growing, particularly given the push and excitement created by ChatGPT’s release into the mainstream. New opportunities for businesses can develop in months or even weeks. However, despite its potential, the field is still maturing and implementing generative AI applications in an enterprise environment will take much measured progress and experimentation in addition to careful investment in resources.
In applying generative AI to business scenarios, companies will need to invest in the infrastructure to ensure content is fresh, secure and protected against hallucinations. As AI makes its way further into more use cases, businesses will benefit from remaining vigilant in new advances and mapping developments to their real business needs.For best practices on using generative AI for your business, watch our webinar “Scaling Customer Service in the Era of ChatGPT.”
Dig Deeper
We’re divulging what our enterprise beta testers discovered when implementing Coveo Relevance Generative Answering in their digital self-service experiences. A must-read if you’re:
- Tired of GenAI companies over-promising with no real product
- Sick of the GenAI noise and just want the facts on what’s actually working
- Ready to get GenAI up as fast as possible – without compromising