Generative AI came. It disrupted. It… left a lot of enterprises scrambling.
Organizations are racing to adopt GenAI. They see huge opportunities (and they’re right!). But, they’re also concerned about their own readiness. And worried about the risks of this new technology (also right.)
About two-fifths of executives surveyed by Google felt a high degree of urgency to adopt GenAI. But, 62% say their organizations lacks the critical AI skills needed to fulfill their strategies. Many also worry about the risks of using these tools within their businesses, with top concerns being inaccurate information (70%) and bias (68%).
Like other disruptive technologies, the initial hype needs to weigh the risks and challenges of business application.
50+ CIOs Reveal Top 5 GenAI Considerations
Because CIOs are in the difficult position of figuring out the best ways to take advantage of rapidly evolving technology… they have unique insights on how to mitigate the risks.
So, Coveo CEO Louis Têtu traveled the world, interviewing more than 50 CIOs to get their best advice.
Here’s what they said…
Consideration 1: Security
This is a broad, nuanced topic. So, we’ll break it down into three specific sections.
I. Privacy of Public Generative Engines
Widely available and easy to use. Public GenAI engines have made it simple for anyone to get seemingly accurate responses from these models. However, this poses a significant risk for enterprises: These public engines save and store chat history to further train their models, potentially exposing proprietary information.
Six percent of employees paste sensitive data into GenAI tools like ChatGPT, security architecture company Layer found. Of those employees, 15% have engaged in pasting data, with 4% doing so weekly and 0.7% multiple times a week.
A real-world example: Samsung Electronics, which banned the use of public-facing AI-generative tools for employees after engineers accidentally leaked internal source code into ChatGPT. Several companies, including Apple and Bank of America, have also banned or restricted the use of public facing engines by their employees.
But, banning something doesn’t mean employees won’t try to use it. The risk of employees entering private and sensitive information – like source code, customer meeting notes, or sales details – into public GenAI solutions remains a significant problem for enterprises.
II. Proprietary Enterprise Data
Public GenAI platforms have parsed the internet. But, they haven’t parsed the unique data your organization stores in popular SaaS platforms like Salesforce, SAP, Sharepoint, or Dynamic 365.
If you want GenAI technology to provide answers about your enterprise’s specific products, services, and organization: that information needs to be indexed. And, that index needs to be connected to every complex system your enterprise uses in a unified way.
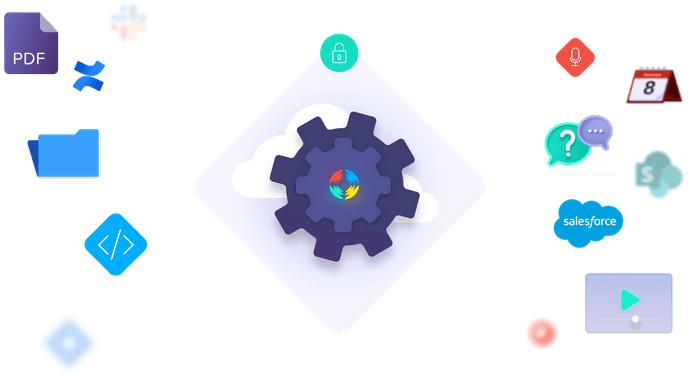
III. Security of Generated Content
Because public GenAI tools don’t have built-in security layers for enterprises, they open businesses up to new security risks. In our recent GenAI Workplace Survey, we found that 71% of senior IT leaders believe GenAI could introduce new security risks in their organization.
If enterprises don’t have a secure index with permissions and roles, generated answers can come back with content users shouldn’t see. For example, let’s say you connect your payroll to one of these unsecured GenAI platforms. You ask, “What is my salary?” The platform could start generating a list of all the salaries in your entire organization. There are no controls for privacy of information.
Consideration 2: Search Relevance
Okay – things are about to get a bit technical. We’re talking about why you need to rethink your humble little search bar – and raise the search bar.
With GenAI, we learned more about Retrieval Augmented Generation, often abbreviated as RAG. RAG is important in helping surface contextual information to LLMs. This in turn generates a more accurate answer that end users can trust — and one that doesn’t hallucinate.
But, not all RAG approaches are created equal. Companies differ in how they set it up and which frameworks they use (i.e., LangChain, LlamaIndex, etc.). This has an impact on the quality of the outcome. Also, RAG approaches often reduce the “Retrieval” part to a semantic similarity search in a vector database. But this isn’t enough, as it won’t provide the most relevant information to the LLM to generate the best answer.
Not only that – nascent RAG approaches miss crucial aspects for the enterprise. Like the full infrastructure needed to manage, standardize, tune, control, and self-optimize search results ranking — at scale and across siloed platforms. Even Gartner highlighted that ‘search augments AI, rather than the reverse’, underscoring the need for strong AI search relevance.
Great search relevance is achieved through a combination of capabilities that, together, deliver a closed-loop, self-learning system. These include, but aren’t limited to:
- Extensive ranking & filtering to ensure the best content is surfaced for end users
- A hybrid unified index that standardizes and normalizes data from different systems with varying file formats — supporting lexical, vector-based and behavioral techniques
- Manual and auto-tuning options to incorporate domain-specific or business-specific content such as acronyms and company-specific product terms
- Advanced security to ensure users only see what they have access to, across varying systems and their different permission settings
- Out-of-the-box connectors and native integrations for popular enterprise platforms, increasing interoperability while augmenting with AI Search & Generative Answering
- Business controls to boost and bury or ‘feature’ select content or products based on business objectives
- Ability to refresh content and data sources at set intervals and easily add new data sources at any time
- Enterprise scalability to ingest and work with millions of documents
- Behavioral AI models that self-learn from user search activity and success which improves the recommendation of content and products, positively reinforcing the entire search and generation system
- Personalization and ability to leverage real-time and historical behavioral information about a user and their activity online to tailor information
- Analytics and insights on model performance to continuously improve digital experiences
Keep in mind – RAG and GenAI are just one of many techniques for helping people find, discover, and get direct answers to their needs. Other techniques are still needed and expected by end users.
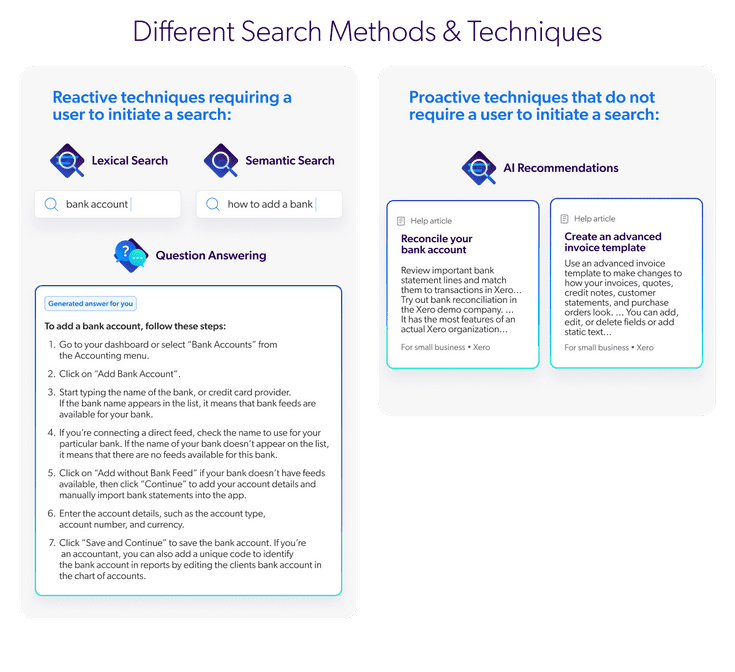
So, what does all this about search relevance mean for you?
Taking a holistic approach and a broad set of capabilities is what will comprehensively transform the performance of digital experiences across your enterprise.
And you can enhance these capabilities even further by investing in a robust, unified AI search platform.
GenAI excels at generating language. But language generation alone won’t be enough if the model doesn’t have an adequate amount of the right content to draw on.
This means investing in an AI search and generative experience platform that can index, categorize, and surface content in a way that respects security, access rights and delivers accurate, trusted relevant answers.
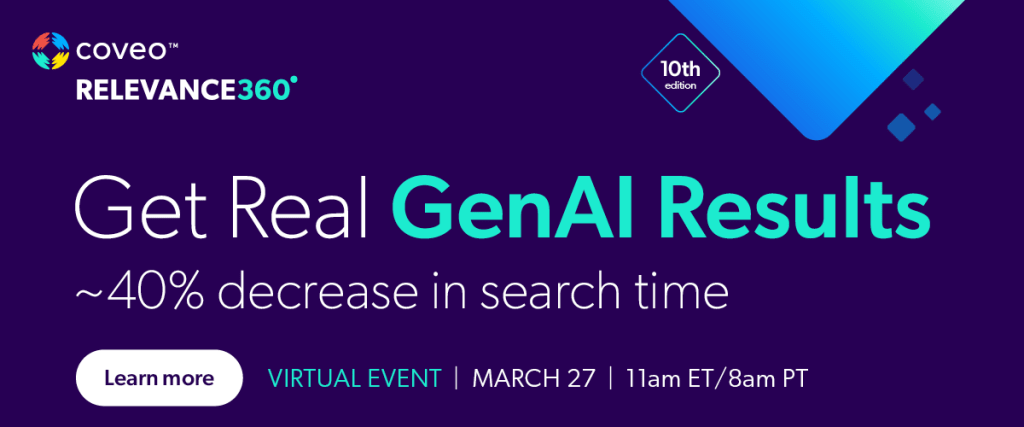
Consideration 3: Yes to Accuracy, No to Hallucinations
GenAI solutions sometimes produce factual errors that appear totally accurate – telling lies with confidence. These are called “hallucinations.”
These are now well documented and one of the greatest obstacles for enterprises when adopting GenAI. They may stem from biases the AI picked up or limitations of its training data.
For businesses, these inaccuracies, biases, or misleading information can have significant negative consequences. Like eroding customer trust and opening businesses up to ethical and legal ramifications.
To avoid these kinds of errors, it’s crucial that you’re able to verify the accuracy of each answer.
Whether generating answers for healthcare professionals or government bodies, companies need compliance and ways to verify the accuracy of GenAI.
You can do this with “grounding”.
In the field of artificial intelligence, grounding is a technique used to create associations between entities for the machine. These chunks are then sent to the LLM, which in turn generates the answer. This real‑time grounding is essential for enterprise applications. It helps to ensure that the output is relevant, accurate, consistent and secure.
You can learn more about hallucinations here.
Consideration 4: Customer and Employee Experiences
AI Search and GenAI need to work together to deliver consistent experiences across all channels. If an enterprise provides both chat and search, customers and employees shouldn’t receive different answers in these channels. This is crucial to offering great experiences.
Salesforce-owned Mulesoft found that 81% of customers believed that one out of five organizations across industries — banking, insurance, retail, healthcare, and public sector — provide a disconnected experience. They fail to recognize preferences across touchpoints and provide relevant information in a timely manner.
Why implement GenAI without first ensuring the conversation you’re having with your customer is consistent?
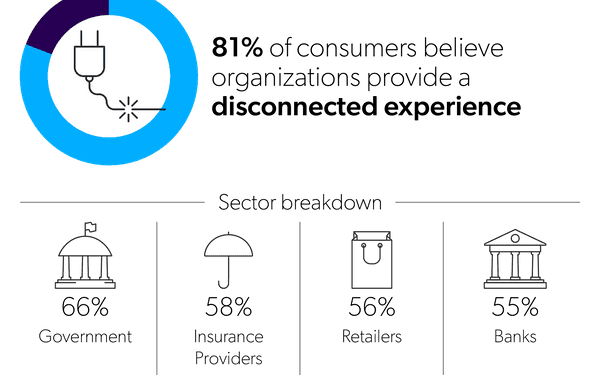
We believe the worlds of intelligent search, discovery, recommendations, generative answering, conversations, chats, and personalization… all converge.
This means you need to raise the bar on search. It’s more than just a box. It’s your window to your customers and employees, enabling 1:1 conversations at scale. It’s your medium for providing smart, unified experiences to visitors: AI-guided recommendations, generative answering, relevant results… All of these have an impact on your end users’ perception of your brand and their decision to do business with you. Or not.
Consideration 5: High Costs
What has the biggest ROI and better experience from an end user perspective: building a solution yourself or partnering with an expert who has one ready?
Well, to start – there are several issues with building this infrastructure yourself. It must contain:
- Necessary security features and role-based access permissions
- An intelligence layer that can disambiguate and understand user intent
- Native connectors into popular organizational platforms
- An API layer that works with any front-end
The expertise and experimentation necessary to build this at scale can take years. This affects both time to market — and having the necessary resources available to innovate.
That’s why leading enterprises are opting for the Coveo Platform™. They’re fast-tracked to results because our solution has been almost a decade in the making.
Xero, a Coveo customer, wanted to improve their self-service and help their users do more on their own. Rather than pouring months of resources into building a solution themselves, Coveo “delivered in a matter of weeks.”
In fact, just six weeks after launching with Coveo GenAI in October 2023, they boosted self-service resolution by 20%. And cut their users’ average search time by 40%. This means remarkable efficiency gains and cost savings.
Getting Enterprise-Ready
Now that you know the 5 considerations CIOs recommend – what’s next?
After partnering with hundreds of global enterprises, we’ve learned that you need platform-based technology. This is the only way you can unify your most relevant content at scale in a secure way.
That content can be fed into your choice of LLM trained on your data. The answers can then be delivered coherently in search, navigation, recommendations, and other customer channels.
Here’s how to do it:
- Securely unify and enrich content across internal and external content sources (content and data layer)
- Generate in-session, personalized search, navigation, content recommendations, chats and conversations (relevance intelligence layer)
- Provide these experiences in any app across a digital journey (engagement apps layer)
Now that you know how to prep your enterprise for GenAI… don’t wait. It’s honestly not that hard when you work with the right platform.
In fact, you can deploy proven GenAI in just 90 minutes – and get ROI within 6 weeks! Coveo customers like Xero are already decreasing time spent searching by ~40%.
Ready to Stop Scrambling and Start Winning with GenAI?
Join our virtual 1-hour event!
AI thought leaders from OpenAI and Forrester will join us to give you:
- Complete strategy walkthrough – How enterprises are getting ROI in 6 weeks with Coveo GenAI
- Exclusive Q&A session – Get all your burning questions answered by the real-deal experts
- Groundbreaking AI Strategy masterclass –
$299FREE hands-on learning with an OpenAI expert