In the world of ecommerce, consumers expect frictionless experiences that instantly meet their needs. Things like one-click checkouts or same-day shipping can no longer really even be considered perks. They are essentials that customers have come to take for granted – and if they aren’t provided, customers will leave to find a business that will.
Notably, this phenomenon isn’t limited to the ecommerce space – the need for instant satisfaction has carried into customer service as well. Customers expect to find the information they seek quickly, preferably without having to pick up the phone.
As a result of this on-demand economy, companies have been forced to quickly assess and adopt technology that enables them to meet this growing digital demand. Wondering where to start?
Here are the five features you need to meet the experiential expectations that customers now carry with them wherever they go.
Intelligent Search
An intelligent knowledge base is one of the best ways to help customers solve their problems on their own, as opposed to contacting customer support. The knowledge base must contain all potential content that might help the customer find an answer to their issue – customers can’t find what isn’t available to them.
In my experience dealing with Fortune 500 companies, I have found that the most sought-after data sources include public documentation, community forums and training videos. Companies tend to think of federating their search as a solution, but that doesn’t usually work in the way that they hope. In fact, it actually complicates the search process, as users do not always know where to find their answer or even what type of content is available to them, and, as a result, promotes case creation.
Rather than federate, organizations should aim to create a unified index and add an intelligence layer on top. This lets you create a unified list of results – and a much better user experience – as they will no longer have to go through different properties to find the answer to their questions.
And the layer of intelligence adds value in a number of different ways.
One of the benefits of adding this intelligence layer is that the piece of content most relevant to a user query always finds its way to the top of the page. Users will no longer need to take additional time to scroll, which is good because they won’t. Think about it, when was the last time you went to the second page on Google?
Machine learning models are able to do this by analyzing user behavior patterns from across multiple search visits to understand which results and associated content lead to successful outcomes. In other words, if a model realizes that for the query X people tend to click on result Y, the model will automatically adjust future search results so that the best-performing content always rises to the top.
Once a model like this is up and running, the question becomes: how can it be scaled for your organization?
Each search interface should be able to send contextual information along with each query it receives. Machine learning can use this information to separate users into different groups based upon search habits.
This is essential because a one-size-fits-all experience is not optimal. Different users may have different intents for the same query, so feeding machine learning in this way is what will enable you to uncover and act upon them in turn. For example, the job department of a user is a good context to leverage since users within the same department will likely consume the same type of content.
Chatbots
Other than your knowledge base, are there sections of your website that trigger multiple questions for customers? Those pages might be good candidates for chatbot use. Certain users may prefer interacting with a chatbot since they need to be guided in their digital journey on your site.
Just like an intelligent federated search page, your chatbot should be indexing content from various sources that may contain information relevant to the end-user. The addition of machine learning allows the chatbot to learn from all interactions with end-users and determine what the best result is on an individual basis.
What results is a self-learning chatbot that can:
- Provide answers without requiring predefined rules or mapping
- Use intelligent content surfacing to deal with unanticipated — or variably worded — queries
- Index content of any source or type
- Preserve full customer context and valuable interaction data that can be carried over to other channels (such as agent-assisted support)
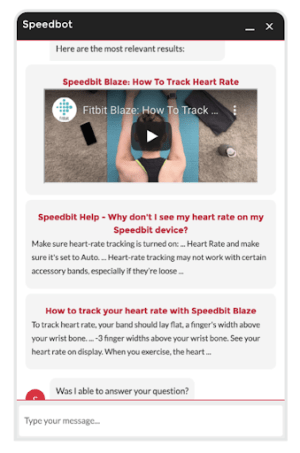
Bringing intelligence into your chatbot allows you to surface relevant content to a given user directly within the chatbot.
The main difference between a chatbot and an intelligent search page is really about form rather than function – the chatbot puts the onus on the end-user to provide additional information which helps the chatbot to filter content.
Personalized Recommendations
A relevant digital experience should also be able to provide recommendations tailored to the individual user to help them on their journey to find the information they need. Organizations that do not suggest additional content to visitors are involuntarily limiting users’ knowledge about your organization.
For example, by not leveraging recommendations, marketers may be missing out on generating relevant webinar subscriptions, as their users are only looking at public documentation and are unaware of what else exists.
Personalized recommendations should be underpinned by intelligence that enables them to learn from your website user page and search navigation history to return the content most relevant to a given user within their current session. Tailoring the digital journey in this way will help users self-serve and increase their product knowledge in the process.
This can be achieved by applying AI and using machine learning models that consider pageview events as the most valuable form of data. This way recommendations will help users understand what piece of content they should view next.
These models should also consider search and clicks by default, but pageviews should remain the core source of truth for recommendations. A different data requirement that can be sent to machine learning models is the user context values. This information allows you to further personalize the recommendations. For example, you could provide different recommendations based on a user’s actions in combination with their role at a company.
Intelligent Case Assist
Not all cases can be deflected. But when a case is being created, how can the process be optimized?
Did you know that cases are often inaccurate, misrouted or incomplete? This type of error from the customer often happens as a result of the case creation process being too complicated for users.
Miscategorization affects case routing which can be very costly to an organization since support agents will need to take additional time to re-categorize the case information and redirect the case to the proper team. This not only impacts the productivity of agents but also harms the user experience, as it will impact the ability of machine learning to provide relevant suggestions while a user continues to fill out the remainder of the case creation form.
Normally, text classification is done based on keyword search and keyword matches, which is what often results in miscategorization. When you add NLP models to the case creation form, they consider the full context – the user’s profile and the information they enter amongst other aspects – to categorize the case. So rather than coming to a static determination based upon pre-defined rules, the models are able to dynamically weigh the importance of certain words and phrases on a case-by-case basis and come to a more intelligent conclusion.
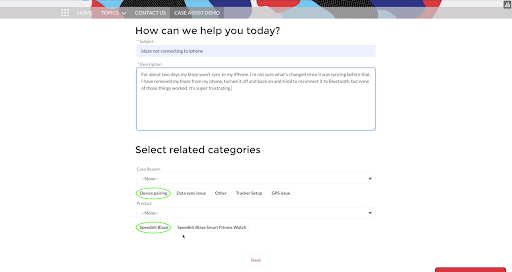
Relevant categories are automatically generated and suggested based upon what the user types in the description box
For example, if a customer is filling out a case about their faulty camera battery, and they also include information unrelated to the issue with the camera – perhaps that it was a birthday gift from their mother who is a professional photographer and loves camera shopping – then the model will ignore the comments superfluous to the issue at hand and focus on what is relevant to the potential case categories available for classification. In this case, the model would be able to hone in on the fact that this person was experiencing a “hardware issue” and did not need assistance figuring out which camera to buy.
This significantly reduces the chance for error in classification and, therefore, the amount of time agents will have to spend to simply get the case in the hands of the person who will be able to solve it.
User Actions
Have you ever responded to a customer only to have them be upset that you provided them with documentation that they had already consulted and, worse, that didn’t help them solve their problem?
This back and forth is not only time consuming but frustrating too. Remember, customers are looking for instant satisfaction. If they haven’t been able to find the answer to their issue before creating a case, the last thing they want is information that has already proven to be unhelpful.
One way to ensure that this doesn’t occur is to provide agents with a detailed list of the user actions performed by the customer before a case is submitted.
With information on what has been viewed and what queries have been performed, agents can provide expert advice to the customer and provide them with information that will actually help them to solve the issue at hand.
Dig Deeper
To learn more about how to transform your customer service experience, read 6 Strategies to Boost Self-Service in Salesforce Experience Cloud.
Ready to experience the leading AI-powered service solution for yourself? Try out a Coveo trial today.