Buy One, Get One. We all know the popular retail phrase. But what if you could: Sell One. Sell Another?
With soaring Customer Acquisition Costs (CAC), almost 5x increase in five years, Ecommerce companies across all sectors are scrambling to figure out how to increase revenue – and margins.
They are looking at ways to increase not only AOV — but Customer Lifetime Value (CLV). The key is giving shoppers a friction-free experience that makes them want to stay and buy more. That has thrust a robust recommendation system into the spotlight.
“The CAC is becoming higher than the average order value (AOV),” reports Pini Yakuel, CEO and founder of customer data platform Optimove. According to Mary Meeker, Internet technologies guru and managing director of Bond Group, calls this pattern “unsustainable.”
In B2C, and now even B2B, recommendation engines encourage website users to buy more because they provide a necessary part of a personalized shopping experience that consumers crave. They also increase loyalty.
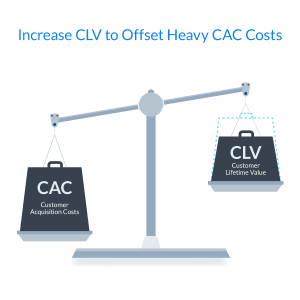
A successful Ecommerce company must have a CLV:CAC ratio of at least 3:1.
Importance of Personalization
In a 2019 Coveo survey of more than 1000 North American retail shoppers, more than 90 percent reported they would return to a site with a personalized digital experience.
And more than 60 percent of shoppers purchased something based on a product recommendation from a retailer, with the number skewing even higher for younger generations: 63 percent of ages 18-24 and 68 percent of ages 25-44.
Other research has found that:
- Almost two-thirds of shoppers don’t mind if a brand saves their purchase history and other information if it results in a more personalized experience.
- A similar number said they would stop buying from brands that aren’t good at personalization.
- 70 percent of consumers are more loyal to companies that they feel understand their needs.
Why Recommendation Engines Increase Personalization
There are different flavors of personalization. Simply saying, “Hi Sarah!,” when I get onto a site, is one level of personalization. Knowing a person’s zip code can certainly help in creating product relevance. Providing a buyer’s history is yet another.
But true personalization means tailoring a journey based not only on previous but current shopping and browsing habits.
This level of personalization requires a robust recommendation engine powered by machine learning. The best ones take a hybrid approach of using both collaborative filters and content-based filters. That means you can even personalize for unknown or first-time shoppers.
Why is personalization so important? The vast choices that make online shopping so alluring can also make it frustrating.Having too many options stresses shoppers out. Recommendations guide people through the overwhelming amount of options they have to choose from, and they simplify their decision-making.
Recommendation engines also enhance the shopping experience, and create sales, by reducing friction and frustration.
Forbes identified recommendation engines as one of the “Top 10 Technology Trends in Retail” for 2020. As Deloitte asserts that “convenience is the new normal” in retail, recommendation engines are an important way to make the online shopping experience easier.
Making Shopping Easier & Productive
Let’s take an example. On the website of a home improvement retailer, one buyer adds the perfect green tile for his guest bathroom to his shopping cart. Many recommendation engines just notice what category the buyer is in – and as a result just serves up more tile, sorted by what was popular with others.
But the buyer has made his selection. He is not interested in more tile offerings. The way this recommendation engine is tuned is not going to add more value, as it is only serving up items that compete with what is already chosen. What you want is a recommendation engine that associates and cross-sells to other categories.
If a person is looking at bath tile, a content-based recommendation engine should understand that a person is interested in additional bathroom items such as fixtures or accessories.
Further, other attributes of that tile included color (green), the brand of tile, and price (within a range). Now, instead of more tile, it would be more helpful for our buyer to see bathroom fixtures and accessories that similar shoppers have liked that included all those attributes.
Why not show the homeowner bathroom vanities, or mirrors? And if one of those items is chosen, what if the recommendation engine could save the shopper (and your support staff) headaches down the line by suggesting tools needed to assemble the furniture?
Recommendation Engines in B2B
So far, we’ve talked about recommendation engines mostly in the context of B2C Ecommerce. They are just as essential, however, for B2B sites. Successful recommendations in B2B come with their own distinctive set of benefits and challenges.
In B2B, many purchases are made by the same customers buying the same items repeatedly. But, just like B2C sellers, B2B sites can use recommendation engines to introduce users to items they didn’t know they needed. The process just looks a little different in this context.
Langevin gives the example of a brand with a few thousand distributors. Those distributors go on the brand’s website regularly and buy the same things, in the same quantities, that they always do.
… to Improve Margins
You can use a recommendation engine that will show the distributors other possibilities for what they can order, such as higher-quality (and higher-cost) related items. Pushing the item with higher margins is another way to both spur sales and even improve margins.
The main challenge in B2B recommendation engines is one of complexity. AB2B seller’s catalog can be a lot bigger and more complex than a retailer’s. That means the machine learning engine has to work even harder to identify relationships using catalog information.
But it may be even more important than in B2C, since many items in a B2B catalog will have little user activity around them that could inform recommendations. For example, a machinery component that costs tens of thousands of dollars might be purchased only a few times a year, vs. a $40 hoodie that’s bought several times daily.
Recommendation Engines Are a Must-Have for Ecommerce
Acquiring new customers is rising at a torrid pace. To stay competitive, sites are slashing prices, offering perks like free delivery, and squeezing margins at every turn. Recommendation engines can help create a better user experience that spurs customer loyalty – as well as provide an essential tool for merchandisers to push higher-margined products.
With recommendation engines increasing both AOV and CLV, they have emerged as one of the most essential investments in Ecommerce. They also are a powerful tool for impulse buying.
Want to start creating more personalized recommendations? Learn more about how Coveo delivers relevance in commerce.
Dig Deeper
Learn more about how to recommend products to first-time shoppers in this 6-minute read.
Predict what people need, before they even know they need it with Coveo’s AI-powered recommender system.