Many things have changed in the last 12 months: after mastering chess and Go, machines learned how to kick your butt at Starcraft too (it also looks like staircases won’t save you anymore); Coveo became a Canadian unicorn (we asked for grizzly bear status, but Silicon Valley notoriously lacks sense of humor); and, in case you didn’t notice, humans now spend most of their days in their pajamas:
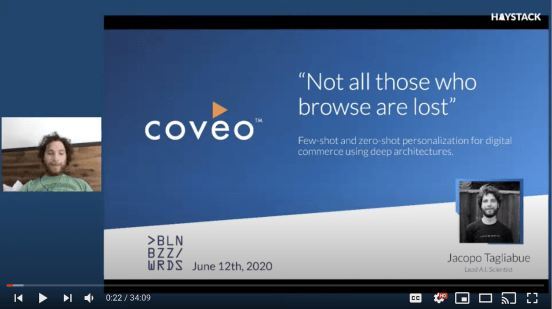
Me, giving a conference talk from my bed (to be honest, I was working in my PJs before you all found out it is cool).
At a time where our softmax function over possible futures looks pretty uncertain, we are happy and proud to share that our AI is here to stay. In the last 12 months or so, our “unofficial outreach” program has created dozens of Artificial Intelligence initiatives, including exciting new features, open source repositories, research conferences, scientific papers, and talks for the industry as well as the general public.
Now, the (not-so-secret) secret about a successful AI roadmap is that ideas are best when shared. None of the innovative work listed above would’ve come into being without a little help from our friends and partners.
In the past year, we have had the honor of sharing the stage with peers from leading eCommerce groups (including folks from, Etsy, Alibaba, Home Depot); we have discussed our work with some of the best tech companies on the planet, such as Google Brain and Amazon; and we have sat on AI committees next to scientists from Bloomberg and eBay.
We took some time in our urban summer to go part of the way down memory lane, collect all the materials we could find from our collaboration with others, and bring them together in one place to share with you.
If I had to pick one thing to be particularly proud of over the course of the past year, the growing network of international collaboration would be my choice. It has given rise to many active areas of research (NLP, recommendations, personalization, time-series analysis, etc.), an innovation-friendly stack, and a “let’s do it” attitude that keeps us all young and curious.
Before sharing some numbers and discussing the next 12 months, I’d like to capture the past year in a slightly different way for the visually-minded humans (and for those finding themselves missing the stylistic simplicity of the early 2000s) – with a word cloud of all our initiatives:
We mentioned we really like models, didn’t we?
A Chart is Worth A Thousand Words
For this post, we collected everything we saved (videos / links / papers / post-its) over the past 12 months related to our industry and research contributions. If you are curious about all the nerdy details, the Appendix at the end should you keep you busy for a while!
In the graphic below, we break down (with the help of our fantastic creative team) all initiatives by use case, tool and geographic distribution. While we did indeed travel far less than expected (given the map), we ended up producing results across a wide variety of use cases. This started with the “obvious” Natural Language Processing and Recommendation, and moved to more exotic topics, like Causal Inference and Intent Prediction.
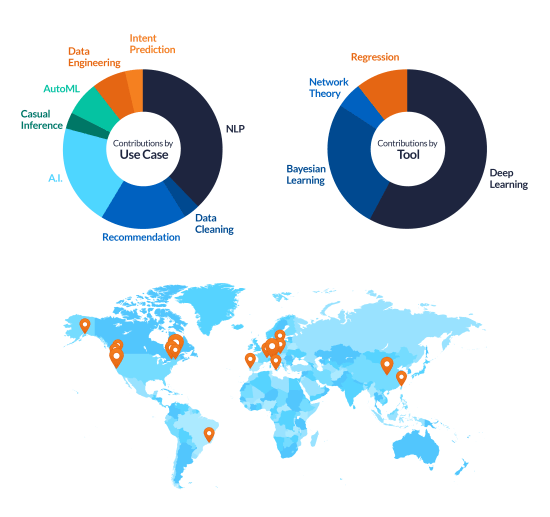
A breakdown of our contributions by use case, by tool, and across a map.
What’s coming next, you ask? Well…
What’s next?
Deep learning is playing an increasingly important role in what we call “applied AI systems”: systems that (to a certain extent) adapt their behavior over time by learning what is “optimal” in a given business situation. If you look at the chart above, you’ll quickly realize that Coveo has made extensive use of state-of-the-art neural networks over the past 12 months – and we will certainly continue investing in deep nets across a variety of use cases.
On a more philosophical note, as aficionados aware of our previous life will certainly recall, we have explored the Deep Learning hype-cycle and some alternative ideas. As awesome as recent progress in neural learning has been, it is also clear that we are still missing fundamental pieces to solve the puzzle of intelligence. Most of our AI tools are still inefficient and brittle relative to other physical machines – e.g. 3-year-old kids.
That being said, diversifying our approaches is a critical imperative that must be informed by science, good risk management and sound business knowledge. Scenarios where data is sparse and pre-trained models won’t do are common, yet our models still need to provide consistent improvement in business KPIs. That is why we are investing in several small-data initiatives, ranging from learning new words to enabling “cold-start” recommendations.
Learning from few examples may not be the sexiest topic out there, but, given that it has broad implications for markets and society, it is one of the boldest. On this note, there are two initiatives for the future we would like to share:
Being part of the AI community means giving back, not just ideas, but also actionable data
When properly aggregated/anonymized, releasing data to the research community is a powerful tool to foster the circulation of ideas and bring attention to neglected use cases. For example, Fashion MNIST was a huge success, and the Rakuten Challenge at SIGIR this year was exceptionally well organized.
Many companies talk about “responsible AI”, and, for us, responsible has a lot to do with reproducible. While we do recognize how hard it is to strike a good balance between openness and competitive advantage, we plan to do our small part and progressively improve the transparency of our research.
Being part of the AI community also requires one to recognize that AI is indeed a fairly old enterprise, much older than machine learning as we know it today
ML in the modern day has strong connections to humans – through linguistics and pragmatics on the NLP side, and to decision sciences on the behavioral side. In the end, when building machines that make humans more efficient – by surfacing the best solution in a support case or recommending appropriate items to buy – we are somehow required to take both sides of the equation into account: machines and humans.
We are working on new models that incorporate insights from other disciplines (such as economics and psychology) to prevent computers from having to waste time learning things we have already known for a while e.g. too many choices are bad, shoppers are not really maximizing anything etc.
We can’t wait to continue our progress towards developing an enterprise AI platform of great depth and truly unprecedented breadth.
In the end, our friend Ludwig said it best:
“You could attach prices to thoughts. Some cost a lot, some a little. And how does one pay for thoughts? The answer, I think, is: with courage”.
To infinity, relevance and beyond!
See you, space cowboys
Whether you are a grad student looking for an internship, a professor looking for datasets and collaborations, or an ML expert looking for the next career challenge, come and join us on our quest to teach machines how to solve practical problems at scale.
Appendix – all initiatives
Research Papers
- Prediction is Very Hard, Especially about Conversion– Paper (KDD19)
- Lexical Learning as an Online Optimal Experiment – Paper (HCOMP19)
- “An Image is Worth a Thousand Features” – Paper (WebConf20)
- How to Grow a (Product) Tree – Paper (ACL20)
- Shopping in the Multiverse – Paper (SIGIR20), Video
- Fantastic Embeddings and How to Align Them – Paper (SIGIR20), Video
- On The Plurality of Graphs – Paper (ECAI20)
- Less (Data) is More – Paper (DATA19)
- The Embeddings That Came in From the Cold – Video (RecSys20)
Industry Conferences
- Few-shot and Zero-shot Personalization using Deep Architectures (Berlin Buzzwords/Haystack 2020) Video
- Efficient Machine Learning for Enterprises (AI Dev World19)
- Less (Data) is More (Global Big Data 19)
Lectures, Meetups and Other Public Events
- “A Rose by any other Name” (University Lecture)
- Why Small Data Hold The Key To The Future Of Artificial Intelligence (University Lecture)
- Learning to Learn (University Lecture)
- Practical NLP Challenges in Multi-tenant, Multi-languages and Diverse Use Cases (Workshop)
- Coveo NLP Challenges in Multi-tenant, Multi-languages Search and Recommendations (Workshop)
- Le Machine Learning chez Coveo, la Recherche et la Recommandation de Produits. (Techno Drinks Sherbrooke Meetup)
- Exploring the Polynomial Space with Probabilistic Grammar(Milan’s Data Science Meetup)
- All’s Well What Ends Well: A Startup Story on Data Engineering and Other Mistakes (Milan’s Data Engineering Meetup)
- Data Scientists as a Service (Bay Area Data Science Meetup)
- Machines that Think Like Us – and Not The Other Way Around (TedX Talk)
- Does the Future Need Us? (TedX Talk)
- AI Panel (JSBA, Concordia University)