High inflation and recession forecasts may be painting a bleak picture of the economy as we’re entering into 2023. But looking at the remarkable volume and quality of scientific research published in Ecommerce AI over the past year can give brands and retailers plenty of hope.
Digital leaders can use investments in cutting-edge AI through the recession to deliver growth and pursue profitability and repeatable financial benefits. But not all AI is created equal — understanding what the latest developments in the field are is pivotal to prioritize and select the right investments.
To catch you up, we have prepared this curated list of the best papers published over the past year.
1. “Does it come in black?” CLIP-like models are zero-shot recommenders
Ecommerce pros will recognize the McKinsey research that found 35% of what consumers purchase on Amazon was driven by product recommendations. Recently, Amazon has replaced those recommendations with lists of ads — but this doesn’t mean recommendations have lost their power. If you’re looking to deliver a better customer experience and maximize profitability, you should look into gradient recommendations.
The result of a fruitful collaboration between Coveo AI, FARFETCH, and Università Bocconi, the first paper in our list was presented at ECNLP5 at ACL2022 and introduced a new type of recommender system in the context of ecommerce, namely gradient recommendations.
Gradient recommendations attempt to suggest products that are closely related to an item under consideration but with a varying attribute such as color or heel height. Given an item (like a pair of trousers), can we find something in the same style, but “shorter” or “darker”?
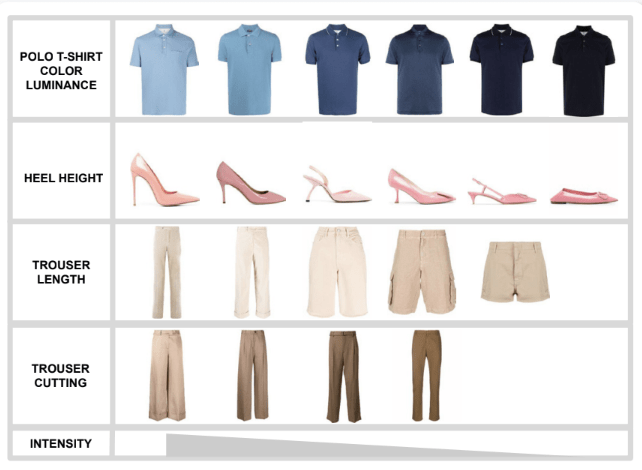
These product recommendations mimic real-life shopping experiences, where shoppers ask sales associates for products that are similar – but different along a specific important attribute.
Here is a demo of this on github. To learn more about all the relevant use cases for eEcommerce recommendations, we also recommend reading our recent ebooks on the Ecommerce Recommendations Periodic Table.
2. Shopping Queries Dataset: A Large-Scale ESCI Benchmark for Improving Product Search
Most frequent e-shopping queries are easy to solve with standard state-of-the-art search engine techniques – and result in near-perfect results. Yet, ecommerce profitability is the elephant in the room that’s taking up more space by the day — clearly there are better ways to serve up results that benefit both customers and businesses.
This paper authored by Amazon’s researchers and published at KDD, introduces and contextualizes the release of difficult, long-form shopping queries dataset – and the related KDDCup 2022 challenge focused on improving product search.
This is an important contribution and challenge. This multilingual dataset encompasses difficult queries like negations (e.g., ‘energy bar without nuts’), parse patterns (e.g., gluten free english biscuits’), and price patterns, among others.
For each query, the dataset provides a list of up to 40 potentially relevant results, together with ESCI relevance judgements (Exact, Substitute, Complement, Irrelevant) indicating the relevance of the product to the query. Each query-product pair is accompanied by additional information.
We praise the initiative of releasing datasets and we ourselves have released datasets to foster innovation in the past years (here and here).
While delivering relevant search results for complex, long queries is critical (i.e., neglecting the long tail often means leaving plenty of dollars on the table), the overwhelming majority of search queries in ecommerce are short, broad, ambiguous, and underspecified, meaning that there aren’t enough linguistic elements to return results that are fully relevant to a shoppers’ intent.
[Research from the Nielsen Norman Group shows the average number of characters is 20.5 for webwide searches. Meanwhile, about 30-40% of ecommerce customers start a shopping session with broad queries like “mens tops”, “nike,” or “handbags.”]
Short head queries provide very limited information about what shoppers are really looking to buy. Does a shopper typing in “shoes” want running shoes, and how do you determine that just from a single word? Does a search for “jacket” mean a winter or a summer jacket, which are completely different yet both relevant sets of products?
This is why here at Coveo we also have focused on a cognitive search approach that guarantees that we can deliver relevant search experiences for long queries, and also highly meaningful results for broad and ambiguous ones.
3. Spelling Correction using Phonetics in E-commerce Search
With more ecommerce searches happening on mobile devices, it’s no surprise that there’s been an increase in needing to serve up results even for misspelled queries. While most misspellings are just misreading or hitting a button twice, there’s a critically overlooked area: phonetic errors.
Failure to accommodate typos leads to poor user experience, zero-result pages and missed revenue opportunities. Presented at ECNLP this year, Amazon’s AI Lab suggests solutions for this ongoing issue.
The English words “blutut sant sistam” mumbled into the speaker of the phone has a similar pronunciation (but nonetheless inaccurate in spelling) of “bluetooth sound system.”
The authors find that this type of spelling error dominates in multiple ecommerce markets with various languages, existing mostly on generic item terms (e.g., “nacklesh” vs. “necklace”) and brand terms (e.g., “scalkendy” vs. “skullcandy”). In this work, the authors present a generalized spelling correction system integrating phonetics to address phonetic errors in ecommerce search.
At Coveo, we agree that typo-correction is a foundational capability that an ecommerce search engine should master. If retailers and brands are unsure as to whether their search is competitive enough, they may get in touch with Coveo and request a site assessment: our strategists and ecommerce experts will audit your capabilities for free.
3. Spelling Correction using Phonetics in E-commerce Search
With more ecommerce searches happening on mobile devices, it’s no surprise that there’s been an increase in needing to serve up results even for misspelled queries. While most misspellings are just misreading or hitting a button twice, there’s a critically overlooked area: phonetic errors.
Failure to accommodate typos leads to poor user experience, zero-result pages and missed revenue opportunities. Presented at ECNLP this year, Amazon’s AI Lab suggests solutions for this ongoing issue.
The English words “blutut sant sistam” mumbled into the speaker of the phone has a similar pronunciation (but nonetheless inaccurate in spelling) of “bluetooth sound system.”
The authors find that this type of spelling error dominates in multiple ecommerce markets with various languages, existing mostly on generic item terms (e.g., “nacklesh” vs. “necklace”) and brand terms (e.g., “scalkendy” vs. “skullcandy”). In this work, the authors present a generalized spelling correction system integrating phonetics to address phonetic errors in ecommerce search.
At Coveo, we agree that typo-correction is a foundational capability that an ecommerce search engine should master. If retailers and brands are unsure as to whether their search is competitive enough, they may get in touch with Coveo and request a site assessment: our strategists and ecommerce experts will audit your capabilities for free.
4. Session-based Recommendation with Dual Graph Networks
Since the majority of ecommerce shoppers are anonymous, the ability to offer personalization and recommendations with little information is critical. This is where session-based capabilities become very important.
A paper presented at the DL4SR workshop hosted at CIKM 2022 focuses on session-based recommendation models, which use the implicit temporal feedback of users such as clicks obtained by tracking user activities.
Two key premises of this contribution are that 1) session-based recommendations are a critical use case in ecommerce and 2) deep learning models have achieved state-of-the-art performance in session-based recommendation. Based on these, the paper then offers a novel session-based recommendation model supposed to perform remarkably well.
At Coveo, we agree on both premises. In fact, our researchers have used an approach based on product embeddings to deliver 1:1 personalized recommendations. Product embeddings have actually become a cornerstone for a considerable amount of machine learning models by ecommerce innovators and rely on deep neural networks. They have been used by the companies that are investing the most in AI innovation, such as Amazon, Walmart, Pinterest, Yahoo, Alibaba, Microsoft, Criteo, and Coveo.
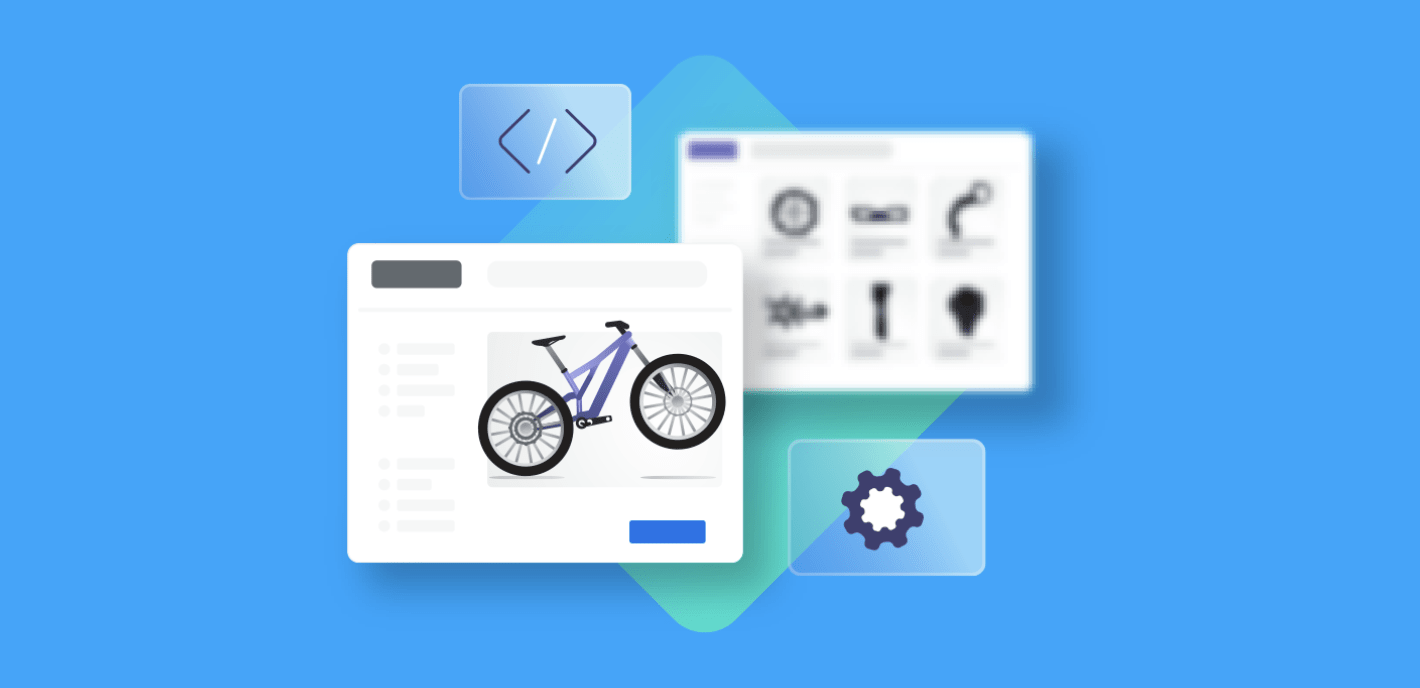
In fact, as part of research led by Coveo, our scientists published a paper (presented at the Web Conference 2022) reporting on the outstanding performance of session-based recommenders using Deep Learning and more specifically Product Embeddings. To learn more about Coveo’s approach and product embeddings in the context of product recommendations, have a look at this post.
5. Addressing Cold Start in Product Search via Empirical Bayes
Just as challenges plague retailers when it comes to “cold start users,” the same issue can be applied to brand new products. Another interesting paper presented at CIKM this year was authored by Amazon researchers and seeks to solve the “cold start” problem in product search.
More precisely, the authors argue that cold start is still an unsolved problem in large-scale ecommerce services. Although cold start has been extensively addressed in recommender systems research, it is hard to find comparable references in the context of product search. Profuse literature on product search addresses related problems such as bias and diversity in search, but cold start has remained a classic topic only in recommender systems research.
We very much agree that common solutions fail to specifically and practically solve the cold start problem in product search. In fact, at Coveo we released Personalization-as-you-go as a specific solution to the cold start problem for both product search and recommendations. You can read more here about its application to product search specifically. This is also why the Coveo Relevance Cloud™ platform was awarded top honors by the UK Ecommerce Awards 2022 in the Ecommerce Innovation category.
6. EvalRS: a Rounded Evaluation of Recommender Systems
What are the best ways to test product recommendations?
To answer this question, it is worth looking at another interesting paper featuring a collaboration between Coveo scientists and researchers from academia (Università Bocconi) and industry (Microsoft, NVIDIA), and which was presented at CIKM.
The paper introduced EvalRS as a new type of challenge, in order to foster a broad, fruitful discussion among practitioners and build in the open new methodologies for testing recommender systems “in the wild.”
Traditional recommendation assessment criteria appeals to two key metrics:
- Mean Reciprocal Rank (MRR) as a measure of where the first relevant element retrieved by the model is ranked in the output list;
- Hit Rate (HR), defined as the ratio between the prediction errors (i.e., model predictions do not contain the ground truth) and the number of predictions.
Behavioral and qualitative tests are also important. And an important aspect to consider when evaluating recommender systems is “being less wrong.”
Not all mistakes are equally bad: if the ground truth item for a movie recommendation is “When Harry Met Sally”, hit-or-miss metrics won’t be able to distinguish between a model suggesting “Terminator” and a model proposing “You’ve Got Mail”.
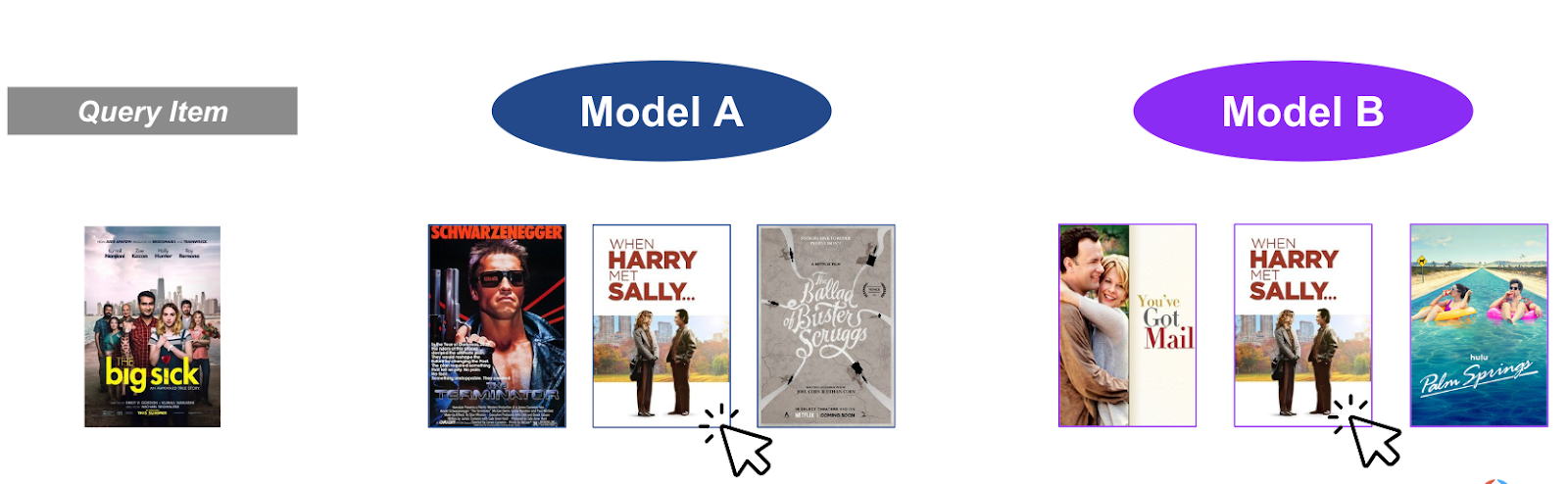
Model A and B have the same hit rate, when suggesting top three movies based on “The Big Sick”, however, the “wrong” movies in the carousels do not provide the same experience: A’s suggestions are way worse than B’s. In other words, while both are “wrong”, they are not wrong in the same way: one is a reasonable mistake, the other is a terrible suggestion, quite disruptive for the user experience.
7. Contrastive language and vision learning of general fashion concepts
The growth of online retail — with one example being one in four fashion transactions now happening online — has made ecommerce a playground for cutting-edge machine learning (ML) models. The downside is that these ML models are often developed for a specific purpose and not generalized enough to be used across industries, which is an unsustainable practice.
This is the focus of another paper based on research led by Coveo and featuring a joint collaboration with Stanford University, Università Bocconi, Telepathy Labs and FARFETCH, was recently published in Nature Scientific Reports.
To help machines “think” like humans, they must be able to master general concepts. The paper shows through extensive benchmarks and novel tests that CLIP-like models can learn an industry — e.g., fashion — and not just “one dataset”. In other words, you can train once, and re-use the model multiple times. This represents a significant advancement in the field, meaning that by leveraging the approach detailed in the paper your ML model can recognize even improbable products such as “long nike dress” (Hint: it likely never appeared in any training set).
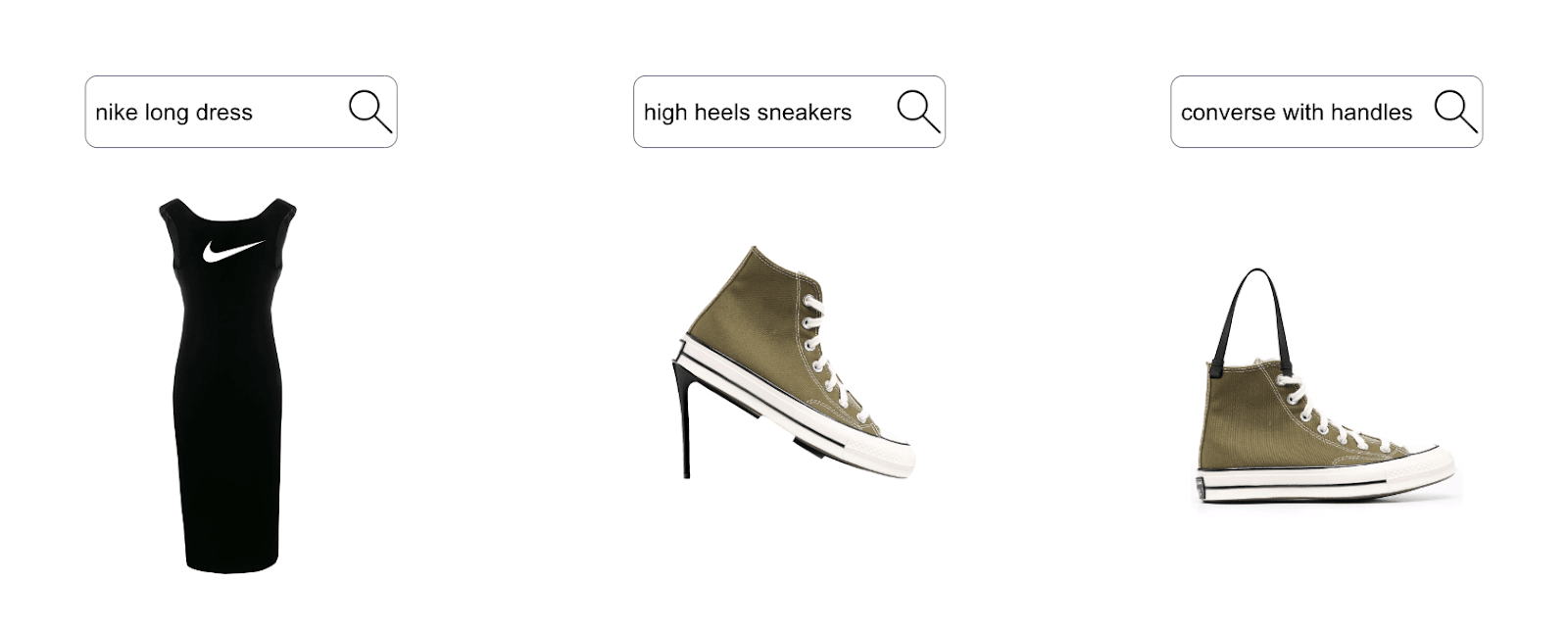
The model was trained on one of the biggest curated fashion catalogs in the world (Farfetch is one of the most successful shops in the world, with more >800k items in store).
8. Multi Armed Bandit vs. A/B Tests in E-commerce: Confidence Interval and Hypothesis Test Power Perspectives
Shaping digital experiences for hundreds, thousands, or even millions of ecommerce visits daily is a tall order, and requires testing even in the best case scenarios. While A/B testing is the traditional method for understanding what experience works better for a specific group, MultiArmed Bandits is a tactic that can address many of the issues A/B testing experiences. How do you know when and where to use these two different methods?
A recently published paper coming from Home Depot’s research lab tackles this dilemma. While A/B testing relies on static allocation, one could opt for a dynamic allocation of traffic using bandits for allocation.
The paper provides a comprehensive, evenhanded comparison between the two approaches, from the perspectives of confidence intervals, hypothesis test powers, and their relationships with traffic split and sample size both theoretically and numerically.
To understand the best practices in A/B testing, you can read more here and here. But on the topic of experimentation best practices, we can jump to our ninth paper in the list.
9. A/B Testing Intuition Busters Common Misunderstandings in Online Controlled Experiments
Surprising results make for memorable headlines, but often aren’t easy to replicate. The biggest selling point of any software, especially ones for experimentation like A/B testing, is trust — but basic statistical concepts are often overblown or misconstrued for effect.
Paper number nine in our list is authored by leading industry expert Ron Kohavi, alongside researchers from Vista and Airbnb. It was presented and published this year at KDD.
Kohavi and his team put on their mythbusting hats, setting to work explaining the misunderstandings with solid statistical reasoning. The paper also provides recommendations that experimentation platform designers can implement to make it harder for experimenters to make these intuitive mistakes.
In particular, we think the brands and retailers should be careful when selecting a personalization and relevance platform, as some might be more prone to encourage biased reasoning and experimental pitfalls. To find out what questions you should make sure to ask personalization vendors, you may have a look at this RFP template that we have compiled at Coveo.
10. Zero party data between hype and hope
The final paper comes from Coveo’s researchers and addresses a hot topic in the context of privacy-aware personalization, namely Zero Party Data (ZPD).
While big data has been a key topic in the business world for years, small data has largely been ignored. At least, until a new flurry of research and spike of interest in ZPD. ZPD was recently popularized by industry analysts from Forrester Research, who defined ZPD as “data that a customer intentionally and proactively shares with a brand.”
This can include preference center data, purchase intentions, personal context, and how the individual wants the brand to recognize her. Surveys have become popular tools to gather information about people’s preferences, wants, and needs. In the example below, customers can express their preference for a specific category.
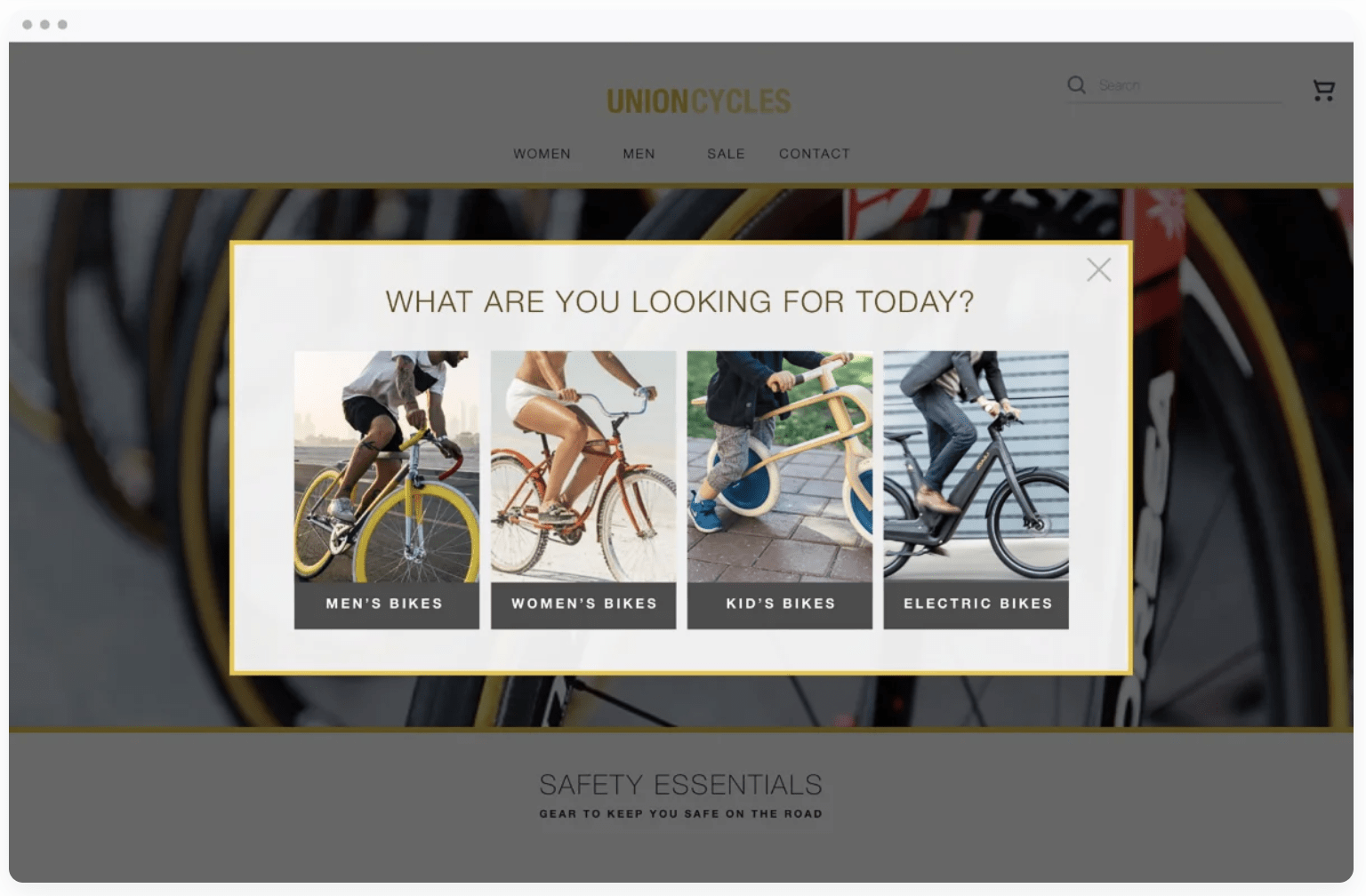
Articles arguing for the value of ZPD to improve personalization and engender consumer trust have appeared in the popular press, business magazines, and academic journals. Advocates of ZPD argue that instead of inferring what customers want, retailers can simply ask them. Provided that the value exchange is clear, customers will willingly share data such as purchase intentions and preferences to improve personalization and help retailers create a picture of who they are.
While the rise of ZPD is a welcome development, this paper authored by Coveo researchers takes issue with the claim that ZPD is necessarily accurate as it comes directly from the customer. This view is at odds with established conclusions from decades of research in the social and cognitive sciences, showing that self reports can be influenced by the instrument and that people have limited insight into the factors underlying their behavior.
This paper argues that while ZPD disclosures are an important tool for retailers, it is critical to carefully understand their limitations as well. The paper also provides a catalog of biases for identifying potential problems in survey design to help practitioners collect more accurate data.
The main implications of this research is that while ZPD can provide a valuable asset, retailers and brands planning to leverage it should complement zero-party data with first-party data. To learn more about effective ways of combining them, have a look at this blog post.