Customer demand for support services will continue to grow at an average rate of 17.6 percent industry-wide and even higher for some segments of the market, according to the 2021 survey Support Transformation – The Guide to Essential Practices and Metrics. It was conducted by ServiceXRG, a consulting firm that helps companies retain customers, grow revenue, and achieve cost efficiencies through technical support and service excellence.
Keeping pace with this rate of demand growth is unsustainable — without changes in processes, technologies, and a modern understanding of support.
We’ll outline all three — so you can create a blueprint for scaling with confidence.
New Drivers of Support Demand
Historically, the top driver of increased demand for support was growth of the customer base — sell more products, get more customers, and support demand grows. Customer growth continues to be the leading driver of support demand.
Modern support organizations do not simply wait for customers to call. Nor do they offer assistance only when asked. Today’s leading support organizations proactively engage customers to prevent issues before they occur and to help customers use and apply products more effectively. Machine learning is key.
Scaling Support Through Staffing
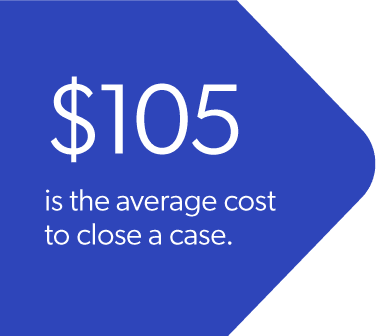
As the customer base grows, so, too, does demand for support. Traditionally, enterprises met this need by adding representatives to answer customer questions on a one-to-one basis. This mode of support can be very effective. But it also costs an average of $105 to close a case, according to the survey. That cost can vary considerably by the type and complexity of product supported, but any way you look at it, traditional customer service is costly.
Fortunately, customers do not always want a person-assisted support session to get the answers they need. In fact, more than two-thirds (69 percent) of customers indicate that when they have support needs, they will attempt to solve the issues by themselves. Less than a third (31 percent) of customers indicate they will request assisted support immediately.
There are two broad areas in which knowledge management and machine learning can assist — by helping customers find what they need and by putting the right information in front of support representatives as they’re helping customers.
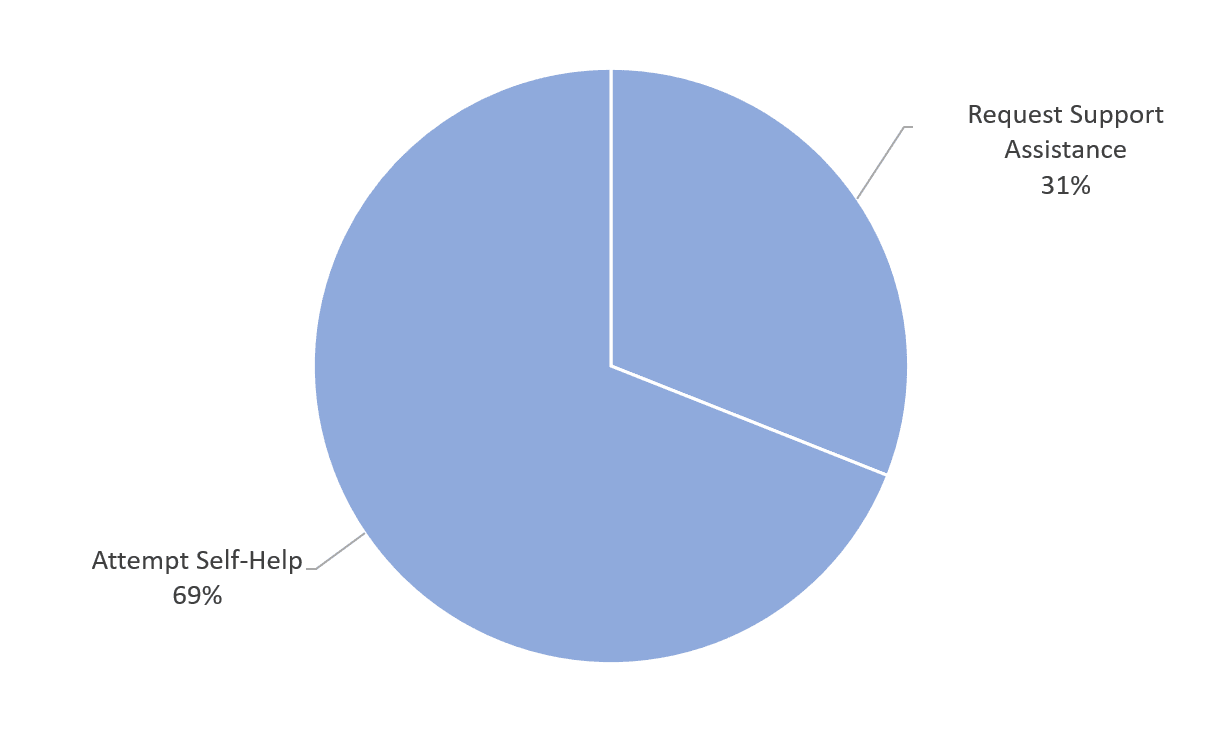
Figure 2: Actions Customers Will Take to Resolve Support Questions (Source: ServiceXRG)
Capturing and Reusing Knowledge for KCS
Capturing knowledge from within the organization is key to both. Increasingly, companies are implementing knowledge management initiatives to capture and refine support’s organizational expertise. The resulting knowledge bases often contain answers to known issues, descriptions about how to accomplish common activities, and insights about best practices.
Enterprises can combine knowledge bases with other repositories of organizational knowledge, including training curricula from learning management systems, answers generated within support communities, product documentation, release notes and other resources. As organizations build these support knowledge repositories, their ability to deliver answers to customers begins to shift from a one-to-one transaction to a highly scalable one-to-many capacity.
Once knowledge is captured, customers access the accrued knowledge of support through self-help channels and automation. In addition, this combined knowledge is available to all support teams.
Machine learning and artificial intelligence leverages this data to serve customers and support teams with relevant information quickly by showing them highly relevant information and products.
AI systems can deliver on the promise of knowledge bases to help solve customer service issues through better search that shows people what they looked for and personalization that tailors the results based on previous activities of the user or others like the user.
Are You Solving the Right Problems?
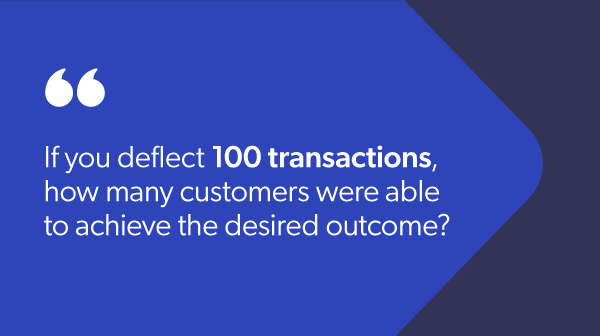
Call deflection, or serving customers through an alternate channel such as a self-service portal or chatbot, is the top metric used by the support industry to indicate that knowledge management and self-help initiatives are working.
Deflection is a quantitative metric that provides no qualitative insights about the outcome of a transaction because it assumes that if a customer finds some bit of information that resolves an issue, then they avoided an assisted service event.
Unfortunately, deflection does not conclusively distinguish between a customer finding something that truly satisfies their need versus them simply going away unsatisfied.
If you deflect 100 transactions, how many customers were able to achieve the desired outcome? Perhaps all, perhaps none – deflection alone does not tell you.
Context Is Key to Delivering Relevant Answers
Customers want to self serve and enterprises build knowledge bases so that they can. So why are we still using call centers? Because search so often still suffers from a lack of context.
Searches that use a few keywords are not sufficient to describe the full context of the customer need, which might include configuration, product version or model, operating environment, past issues they have reported, or any other revealing details. Further, the customer will not likely know if there is an answer or if they simply search for the wrong terms.
But machine learning can deliver content that serves people in very personal ways. It can use signals, such as where a person is geographically or what they’ve clicked on as they move around a site, to anticipate what a customer actually needs. These signals provide context for the search, which leverages the knowledge base in ways that search alone cannot.
Delivering on personal expectation means that the customer will have a higher degree of confidence that they received the answer they needed and will not seek an answer elsewhere.
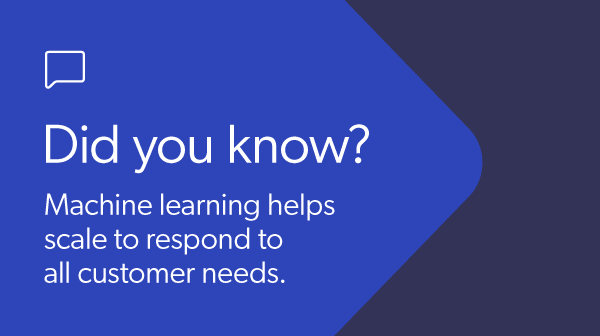
Customers Aren’t ‘Deflections’
Machine learning increases the return on investment that companies spend to develop their knowledge bases and assures that customers can realize the value from our products and it helps scale to respond to all customer needs.
When customers can use and apply our products successfully, we know that there is a lower likelihood of churn and a greater likelihood of sustained and profitable customer relationships. We know that we delivered the right answer when we:
- Removed an obstacle that inhibited a customers’ ability to use and apply products.
- Presented guidance, insights, and best practices to accelerate customers’ ability to adopt a product and achieve goals.
- We reduced a reason that may otherwise compel a customer to churn.
- Increased product adoption usage rates.
- Retained or expanded recurring revenue from existing customers.
Deliver Relevant Answers and Outcomes
The opportunity for support to impact customer relationships is significant. Support must become more proactive and engage with customers to fix issues and to help customers apply and succeed with our products.
Support can only engage in high-value activities if it can scale. To scale, support must leverage its knowledge to deliver answers to customers without the direct involvement of support staff. Most importantly, for support knowledge to be impactful it must be found and applied by customers.
Intelligent search platforms and automated tools (e.g. support chatbots) must be intelligent enough to understand customer needs and the context of these needs. Through machine learning, search platforms can establish the necessary context to deliver the right answers to customers when they need them.
When we focus on helping customers to be successful with our products, they will make us successful. Support outcomes and results cannot be measured simply by transactional metrics such as time to resolve, case volume, or level of deflection. Support must focus its efforts on the retention and expansion of customer relationship value.