Maybe it’s the hype around digital transformation coming to fruition. Maybe it’s the lasting effects of the global pandemic. Regardless, the move toward richer, more contextually relevant digital self-service experiences is in full swing.
How modern enterprises are delivering on this demand is the topic of an upcoming conversation in American Eagle’s Sitecore Watercooler podcast with host John Price and his guest, Coveo’s very own JC Dumont. The duo discusses some of the compelling ways self-service is evolving in light of new use cases for search. Having tuned in early, I’ve put together a look at some of the more compelling topics.
Self-Service Really is Ubiquitous
Today, self-service expands into all areas of life and business. “Good information sharing,” a core tenet of self-service, has broad reach and myriad use cases. Take Coveo as an example: the platform started as an enterprise search solution to help internal employees get answers on their own. Today, that search has evolved to do the same in public-facing self-service portals, communities, and agent experiences—all of it powered by artificial intelligence and machine learning (ML).
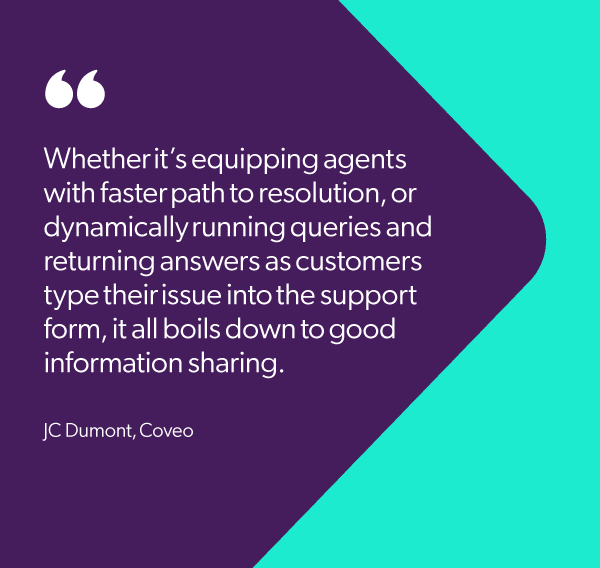
“Whether it’s equipping agents with faster path to resolution,” says Dumont, “or dynamically running queries and returning answers as customers type their issue into the support form, it all boils down to good information sharing.” The challenge is doing all of this at scale.
The Information is Already There
For many enterprises working toward better self-service experiences, a lack of rich information isn’t the problem. On the contrary, most are sitting on vast amounts of knowledge that might be useful to customers, employees, and other knowledge “consumers.”
The struggle lies in delivering the most relevant info to the user based on their context (language, location, device, and so on). As Dumont points out, building your own search logic with this level of coverage and depth is complicated, to say the least.
“A lot of clients try search out on their own using open-source solutions, such as Apache Solr. What they find is that a search experience based on hardwired rules and logic is hard to build, difficult to maintain, and a bit too inflexible.” He adds that most teams don’t want to—or cannot—train in-house staff to adequately code, master, and maintain an open-source search solution. This can lead to gaps in coverage that make it difficult to scale self-service in the long run.
Machine Learning Can Proactively Fill “Gaps in the Stack”
Instead of constantly scrambling to identify gaps in the search experience, ML-powered search can help detect these automatically and serve up what’s best to ensure an ideal outcome. And it can do so without requiring human input from, say, administrators on things like search tuning or rule building.
In the end, a ML-powered search platform can automatically consider and learn from all kinds of user interactions—what they navigated to, clicked on, searched for, etc.—to improve the quality and relevance of self-service experiences.
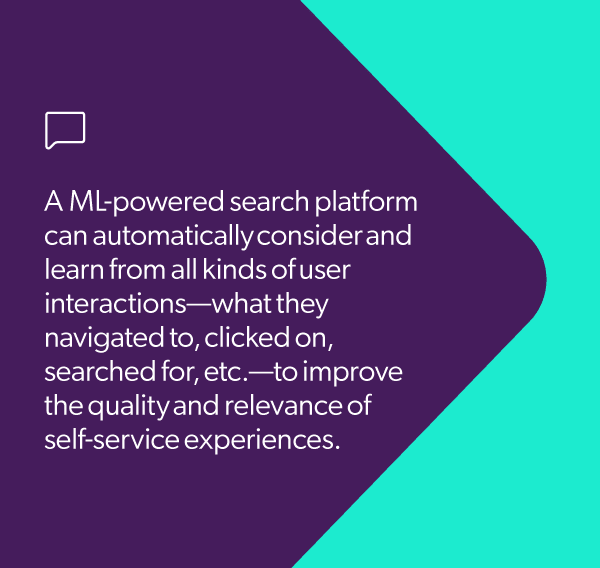
The Ecommerce Crowd is Catching On
This was one of the more eyebrow-raising moments of this interview. We know that people have been forced to focus on digital experiences, especially because of the pandemic. Notably, this includes ecommerce, where search-powered self-service experiences are finding a home.
It makes sense at a fundamental level: think about how you shop online for something like tennis shoes. If you’re like many consumers, you’ll type the shoes you want into the search bar. You might type in a particular brand, style, or color. The sooner that search experience can connect you to what you want to see, the closer you are to a purchase decision.
ML search can deliver this kind of relevance by automatically serving up better search results based on the signals the customer is sending. “In one ecommerce project,” recalls Price, “Coveo ML was improving conversions and average order value by accounting for all of this nuance.” If that ecommerce platform is built on Sitecore, all the better thanks to the Coveo integration.
It’s All About “Stitching” Experiences Together
As we’ve seen in the ecommerce space and elsewhere, a relevance engine built on ML can be incredibly powerful and remarkably scalable. Now, enterprises want to take it a step further. They’re asking, “how can we apply a solution like Coveo beyond just our website or community?”
These organizations need to “stitch” together a lot of different events with a single search platform and experience—“relevant experiences everywhere,” as Dumont calls it. What you learn on your website from ML can be applied to make support experiences smarter and richer elsewhere (in Salesforce Service Cloud, for example). Based on data and insights generated in different events, you can connect the dots and improve the overall experience for everyone.
Consumers Put Search First and So Should You
Another reason that some organizations struggle to get the most out of their search-powered self-service experiences is timing. As Price observes, too many organizations undergoing website projects, redesigns, or restructuring leave search until the end. His advice? Make finding and implementing search a priority from the beginning.
After all, search is what consumers themselves prioritize, isn’t it? Whether it’s B2C, B2B, or ecommerce, people turn to search first to find info immediately. To get to the outcomes you want, you need to enable customers and employees to do more with each and every query.
To listen to the full conversation, meet us at the water cooler and tune into the podcast.