Is your ecommerce store making the most of context based recommendations?
I’ve been shopping for a graduation gift for my niece, and it’s been a frustrating experience.
Aside from money, I have no idea what college graduates are looking for. It’s almost enough to send me back into a physical store so I can have someone help me. It’s also made me wonder why more retailers don’t use recommendation engines.
On digital channels, recommendations are equivalent to great brick-and-mortar sales clerks. They save time, make it easier to find things, and showcase items that other people really, really like — and all of that increases purchases. More importantly, machine-learning-driven recommenders will help you reach a variety of Key Performance Indicators (KPIs). And those don’t only impact your company’s bottom line — often your bonus, too.
If you’re a merchandiser looking to implement AI-powered recommendations — or you have them running and you don’t like the results — you might be interested in our free ebook, The Recommendation Periodic Table.
In it, we break down the 17 essential recommendation elements of an ecommerce website that fit into five different categories. In this blog, we’ll take a closer look at one: context based recommendations.
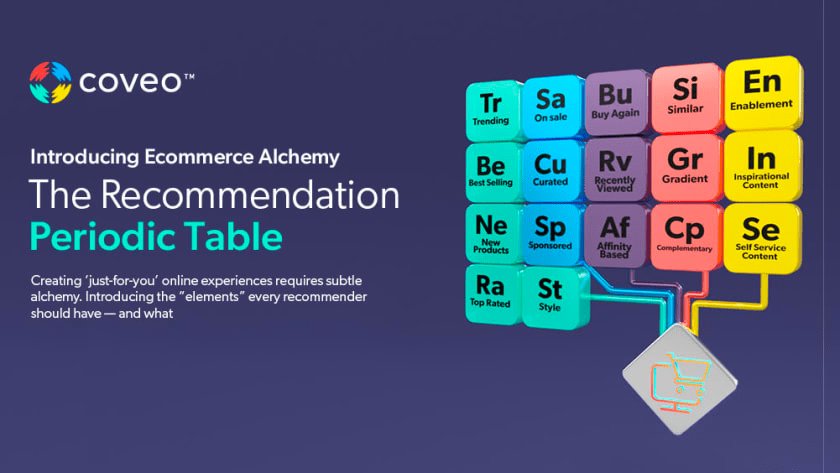
What Are Context Based Recommendations?
Context based recommendations are a family of five different elements. They are suggestions based on a few different things: Product characteristics, what you know about customers themselves, observed retail trends, and other people’s behavior.
Think of context based recommendations as what’s trending, what’s relevant,and what’s new.
They’re terrific if you know your shopper — and equally great for guest shoppers, too.
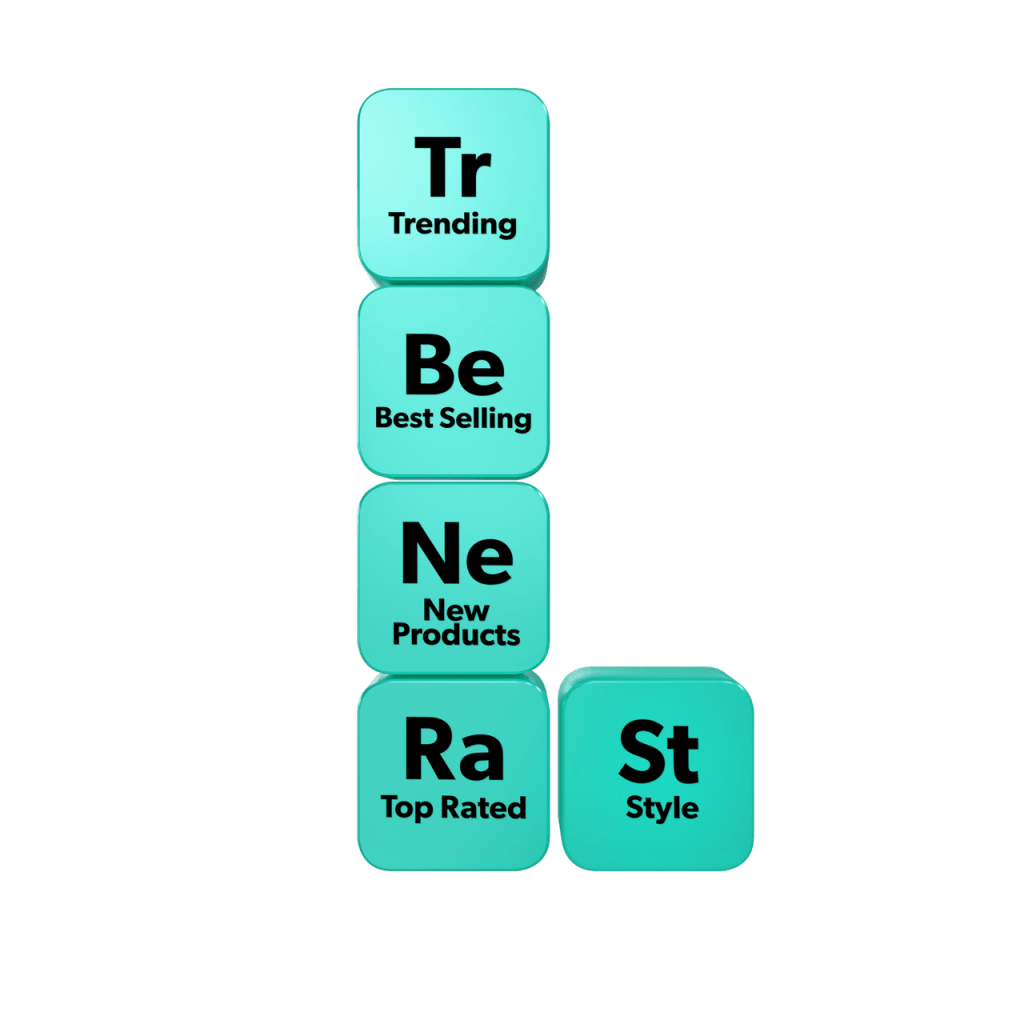
Each of these recommenders has a variety of data at play that are weighted/influenced by machine learning. In particular, you will find that context-based recommenders will improve your click-thru and conversion rates.
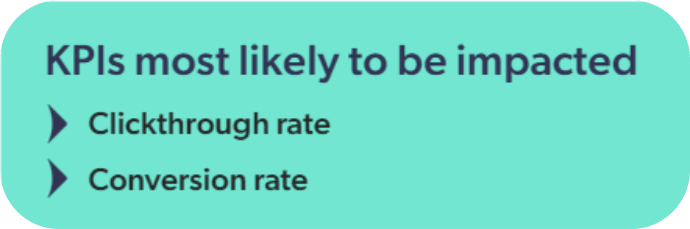
Recommendations Arise From Actual Product Interactions
Trending & Best Selling
Let’s talk about just what these context-based recommendations are in real terms – because some of them can kinda seem like the same things. The first two we will look at are Trending and Best Selling.
Trending recommendations showcase your most sought-after products that are selling right now. These differ from best sellers, which are the products you’ve sold the most over a period of time.
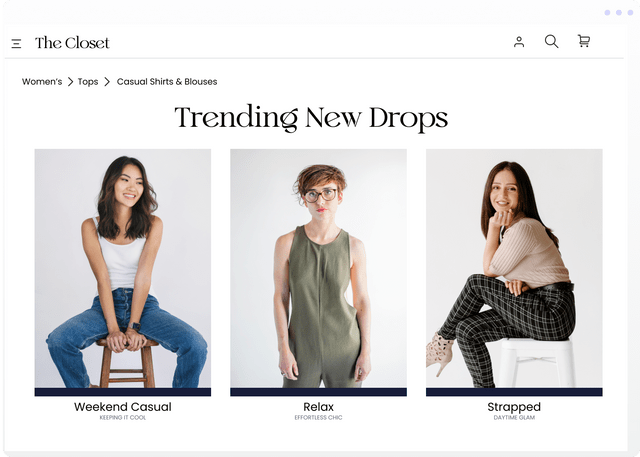
They are not mutually exclusive, meaning your hottest selling product on a certain day may also be a product you’ve sold a lot over time.
And you can adjust best-sellers, depending on seasons, but trending items change from day to day. For example, you’ll want to highlight your best-selling swim suits in April and your best-selling snow suits in November.
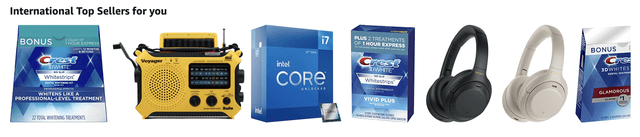
New Products & Style
There is also a bit of overlap in New Items and Style recommendations. New items are exactly what they sound like — items or services new to your store. Shoppers often like to see the latest thing prominently displayed on your site — and they may know what new items are before arriving at your shop. For example, new smartphones and other electronics often get a lot of media attention before they go on sale. Those items you definitely want to put front and center for shoppers.
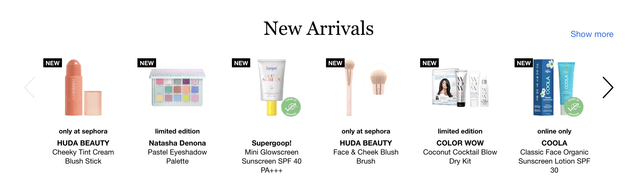
This recommender is also ideal to solve the cold product problem. New products sometimes suffer — and are suppressed in product ranking — precisely because they are new. There’s no customer history associated with these products, making it difficult to understand how to relate it to other items in your catalog.
This element along with style recommendations are terrific in fashion. This lets you showcase and suggest items that complement a product someone has searched for or clicked on. Think about showcasing an entire outfit or a watch that would look great with a certain jacket.
Style recommendations can also begin with the retailer when you show the latest styles in carousels that showcase casual footwear, luxury items, products with easy returns, or items by specific brands.
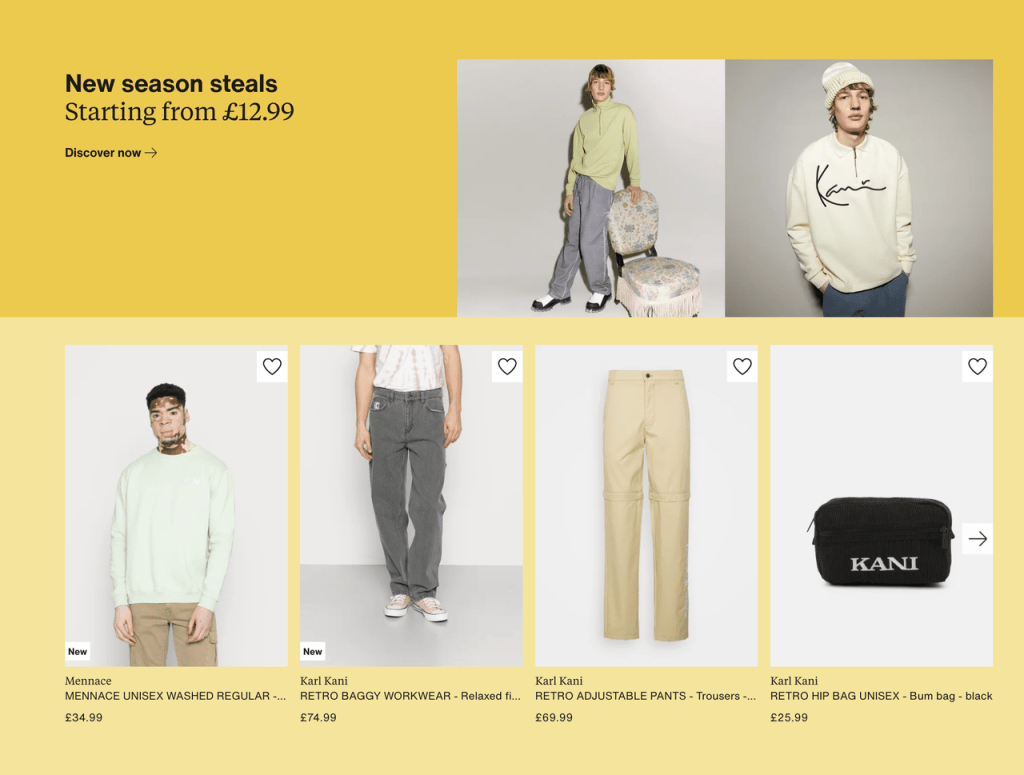
Top Rated Recommendations
The final element is: Top-rated. This recommender is terrific for shoppers – who get independent opinions about product quality and usefulness – and retailers – who see a reduction in returns and negative customer experiences.
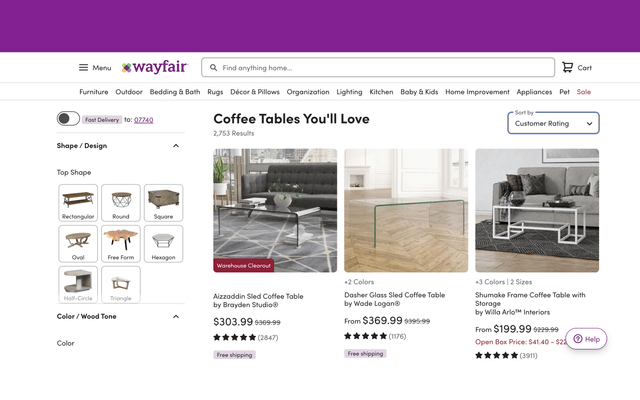
The important thing to remember about all five of these recommendations is that they save shoppers time and effort. They also build excitement around their shopping experiences.
Shoppers can find them without having to search your site and without having to think about the specifics of what they want – because it’s right there for them to see.
This is especially important because consumers spend milliseconds on a site before they form an opinion of it and spend only about 5 minutes on average on a retail site.
Recommendations Are Personal
Recommended products validate shoppers’ decisions and add a social proof element to the recommendation, implying that since other people are doing it so should they. They are intended for new customers or even returning customers that may be influenced by what’s trending right now.
These recommendations are generally best used on homepages and can be even more effective if geo-targeted according to shopper location — after all, people in different locations buy different things. For example, someone in Miami won’t be interested in snow shovels, while someone in Boston wants to know what the most ergonomic snow shovel is.
You need to meet the wants of a growingly diverse group of shoppers, so your sites need to be flexible enough to provide an array of tools — without overwhelming any one shopper. Think of it as having a personalized site for every shopper who visits.
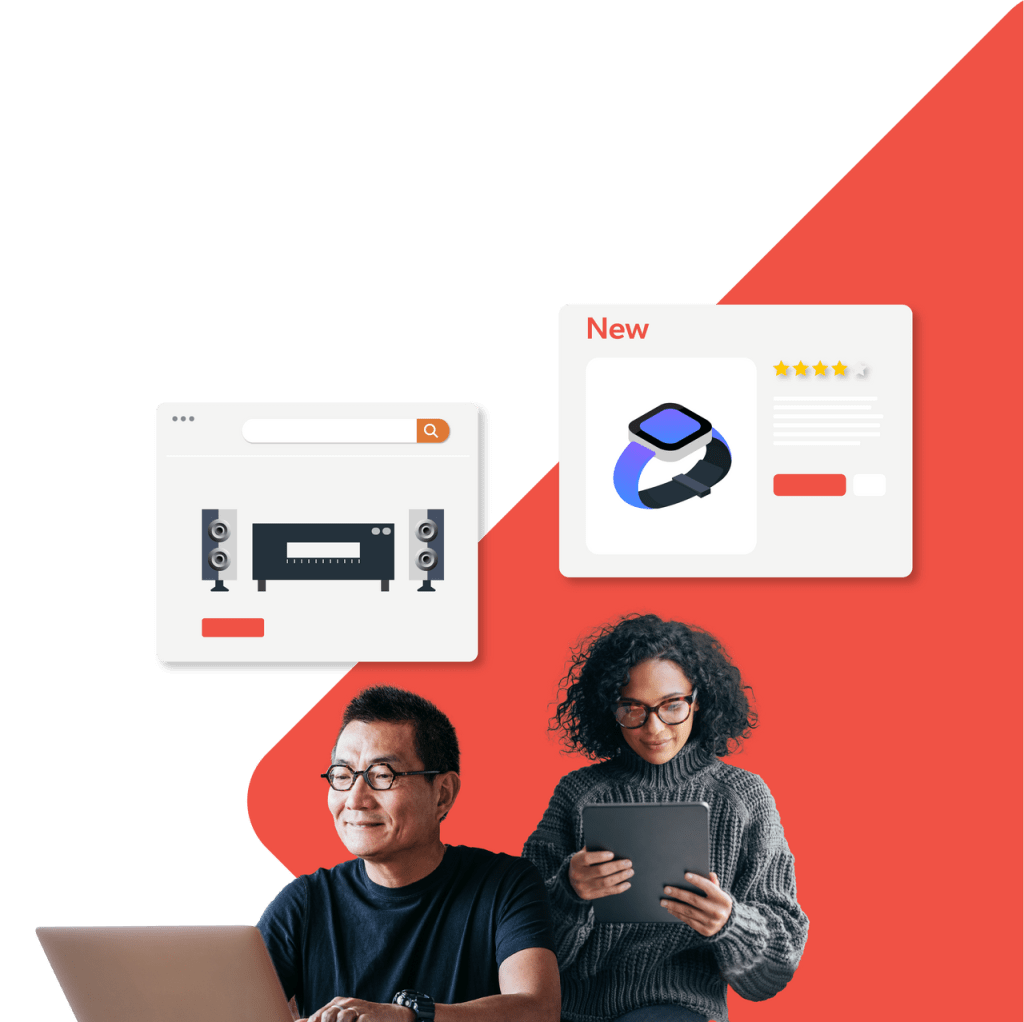
This is especially true of fashion, which reached almost $1 trillion in 2022. Our survey of 4,000 adults found that 50% of shoppers buy fashion online. But what’s fashionable in one place isn’t in another. And trending is especially important in fashion.
People abandoning websites without purchasing because of a lack of hyper-personalization, or 1:1 personalization, is on the rise. While only 6% last year, it more than doubled to 14% — and is likely to continue increasing as shoppers become more aware of how easy it makes it to shop.
Shoppers, especially in the growing Gen Z segment, are motivated by what others like them are buying. They want personalized shopping experiences underscored by recommendations that highlight hot, bestselling, new, and top-rated products as well as those with matching styles.
Give Your Shoppers With What They Want
Personalization is knowing who someone is when they get to your site. Hyper-personalization is knowing what they want when they get there.
If that sounds like you might need a crystal ball connected to your website, let’s just say that you might know more about users, even cold-start ones, than you think. Your recommendation engine can use the following types of data to create context:
- Geography
- First-party data
- Historic data
- IP address device
- Order data
- Margin data
- Time of day
- Behavioral data
- Product attributes
- Real-time
Having a site that shows consumers what they want before they know they want it is a powerful way to build brand loyalty.
Take Control of Recommendations
Robust search with Artificial intelligence and machine learning can help retailers use this data not only to personalize the shopping experience but to make their sites perform better for each customer — no matter what that person wants to see.
With all these recommenders, it’s important to know that you should be able to weigh the results based on business metrics. Layer on margin data or inventory data to promote the products that make the most sense to your bottom line.
Find a recommendation engine that allows you to draw attention not only to trending products but hot sales and/or seasonal products that would hold the most value and revenue during select parts of the year. Coveo provides a merchandising hub for you to control all product promotions – without the need for Jira tickets to your IT team.
Dig Deeper
Wondering what else goes into creating a personalized experience for each unique shopper? Check out our free white paper, The Ultimate Guide to Personalization in Ecommerce, for all the details.