I recently attended a Consortium for Service Innovation team meeting on Predictive Customer Engagement. A new model is brewing with the Consortium that is once again, extremely compelling and current. The Predictive Customer Engagement model begs the question “How can we provide information that we have, that customers would value, but don’t know how to ask for?” What this translates into for me is reducing the effort of your customers and even your customers’ customers.
Reducing customer effort means making self-service easy, relevant and personalized and delivering that via a consistent customer experience. Leveraging data from previous positive self-service experiences enables us to become more proactive at improving future ones. The data being captured should be broad and deep enough to gain the user’s trust that we are presenting them with the most relevant information at that point in time.
First we need to understand the foundational principles of predictively engaging customers. One that is at the top of the list is emotion. In exploring some examples of predictive customer engagement, a common theme is understanding the emotional response elicited from the customer after the experience is over. While not always positive, emotional responses are required for customers to perceive a higher value which leads to an increase in trust and eventually customer loyalty.
For example, I subscribe to weather alerts on my phone and get notified when there is a storm in the area. What if I also subscribe to alerts from my business system vendor and get an alert to proactively check the backup schedule to ensure it runs successfully in case of a power failure? Or, taking it a step further, what if the vendor sends me an alert that it already performed the backup in anticipation of a future power failure based on the weather report? Would that elicit an emotional response of relief, trust and appreciation? I believe the answer is yes.
To apply this to your business, one may quickly become overwhelmed at the volume of data required to analyze and make accurate predictions. Machine learning makes this much more accessible and can quickly exemplify the intelligence of a company to its customers when relevant, timely content is presented in the right context – especially if it is done before a question was even asked. The beauty of this is that the intelligent behavior improves over time. The more data and user behavior and effective outcomes are measured, the better we become at doing it.
None of this is possible, however, if we don’t have the knowledge in place and in a form that can be easily consumed by our customers. Many of the companies I speak with have hundreds of knowledge interactions documented, but have them hidden from customers because they are waiting in the queue for a technical writer to review and publish. This takes us to another important foundational element of an intelligent customer service experience: timeliness. The value goes to zero if knowledge is shared too late. With all of our power to predict, analyze and present data from machine learning we still need human intervention to create timely, relevant and compelling content. To do this, you need a structured process to create trustworthy, consistent and consumable content.
For the content to be available, we recommend following industry best practices as outlined by Knowledge Centered Service v6. There are several underlying core principles of Knowledge Centered Service v6 but one that ties to the required structured process mentioned above is the need to “recognize that knowledge is the product.” We need to focus on creating a knowledge base to capture the collective knowledge of all knowledge workers in order for it to be leveraged by the machines we know will do great things. We also want to make sure we work smarter and focus on capturing knowledge that is new and reuse what is known. This increases our effectiveness, productivity and capabilities to focus on proactive activities rather than reactive ones.
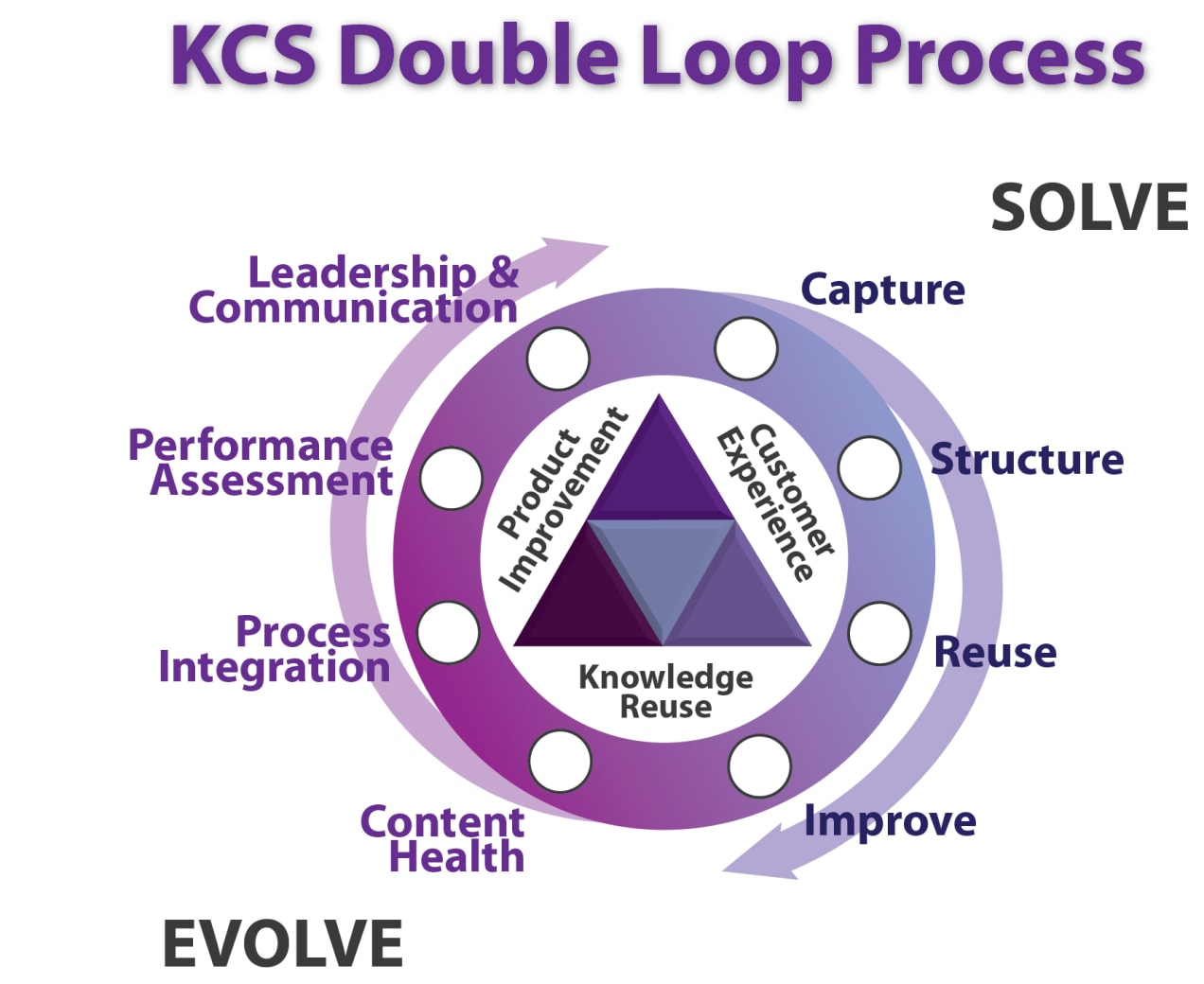
Source: Consortium for Service Innovation
Once we have the knowledge capture process in place, we can then focus on learning from our customers and improving our knowledge ecosystem. Once the knowledge factory is pumping out new knowledge structured for use, we can then start to analyze usage patterns and become more predictive and proactive. Putting these processes and programs in place is not easy but when you have a team that has hands-on experience implementing successful knowledge programs like Coveo does, it makes it much easier.
Join our webinar this week to dive into more of the details while we take you on a knowledge improvement journey. The benefits far outweigh the risks and the return, if done right, is huge.