With Coveo, Mathematica Policy Research has taken website visitor experiences to the next level.
The countdown is finally coming to an end: in just a few weeks, Google Search Appliance (GSA) will be sunsetting forever. Since Google’s initial announcement in 2016, brands have explored solutions for not only Google Search Appliance Replacement, but to upgrade to a self-learning insight engine which caters to today’s tech-savvy website visitors. We would like to highlight one particular organization that has already seen success from transitioning from GSA to our cloud-based insight engine, Mathematica Policy Research, a New Jersey-based think tank.
Seeking relevance
With its organization-wide mission is to improve public well-being through data, Mathematica saw the sunsetting of GSA as the perfect opportunity to rethink the way it could deliver a more relevant experience to their internal and external website visitors. Despite having a large advanced search box on their sites, many users weren’t aware of this functionality and those who used it frequently were frustrated with the lack of relevant results their queries were generating.
By making the switch to an insight engine, Mathematica was able to overcome these five challenges:
1. Limited search usage analytics
GSA had minimal search click tracking capabilities. Without a way to process and visualize the data, it couldn’t assess positive or negative outcomes of a query. Mathematica’s new AI-powered search changed this experience for web administrators and content managers by providing them with rich search usage analytics fit to drive business-critical KPIs. The machine learning component not only understands user behavior and context, but helps to improve user adoption. It can also learn the content that is needed to fill gaps and identify the best content while automatically optimizing relevance of search results.
2. Inability to A/B test
A/B testings helps brands avoid unnecessary risks by allowing them to target their resources for maximum effect and efficiency, which in turn helps to increase ROI whether it is based on short-term conversions, long-term customer loyalty or other important metrics. Any changes on GSA took effect immediately and globally, which had made it very difficult to perform A/B tests. With Coveo, Mathematica is now able to test out search relevance at any given moment.
3. Manual effort to boost relevance
GSA would score content based on predicted relevance, but its accuracy, effectiveness and efficiency were nowhere comparable to Coveo’s machine learning component which optimizes search results relevance based on user search behavior. In short, it looks at end-users queries and search result click behavior made over a given period to constantly learn what the best search results for each query are. When a learned query is performed again, machine learning recommends the best learned search results by boosting their ranking score so they appear among the top results.
4. Limited query and indexing pipelines
GSA offered relatively few ways to alter the query or indexing process. It often required hacks like inserting a proxy in front of the web crawler or the query API. Coveo AI-powered search and recommendations allows one to create custom filters in the query pipeline so that specific content would be searchable and matched to specific audience groups. Mathematica implemented this successfully through indexing permission for appropriate audience groups: internal vs external.
5. A rigid and immalleable user interface
Modifying GSA’s XSLT-based UI was tedious and difficult. Mathematica’s new open source JavaScript Search Framework allows the organization to create feature-rich search interfaces. Mathematica was able to easily deploy and customize a feature-rich, client-side search interface in any of their web pages on their sites to surface from a cloud index. The framework is essentially a block of HTML and CSS defining a layout to organize a set of components. Components such as search box, facets, tabs, or result sort options are used as building blocks to define your search interface.
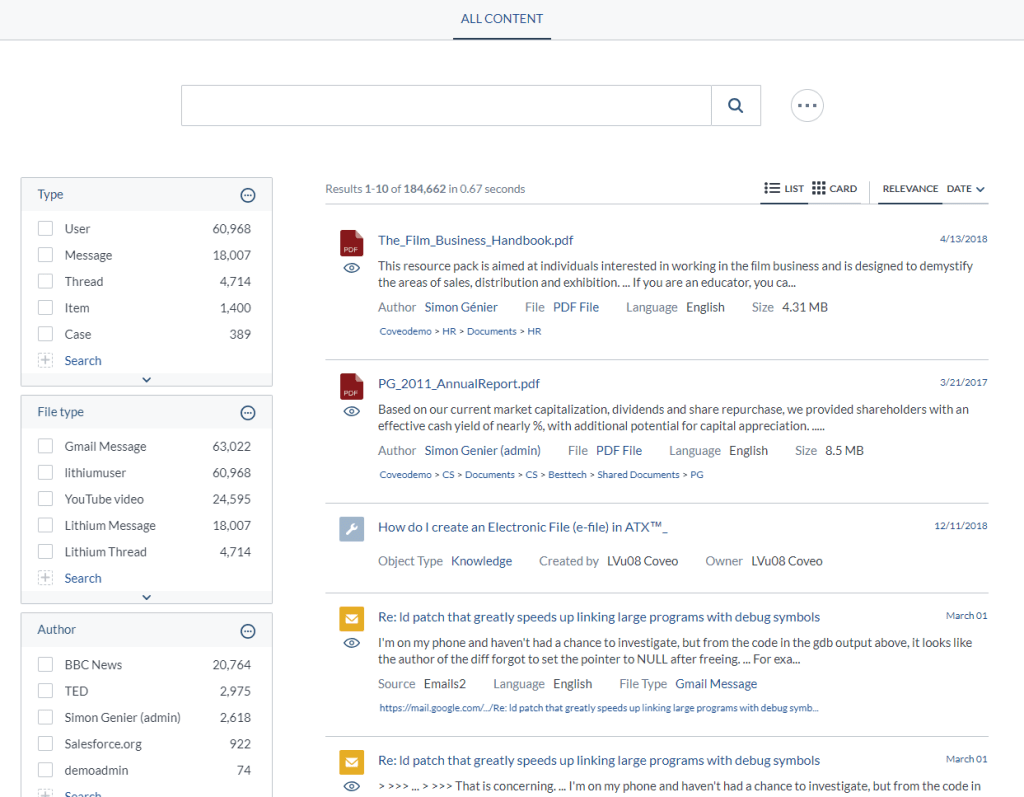
Mathematica successfully implemented Coveo in August 2018, taking web visitor experiences to the next level. The results have been striking: the new AI-powered search and recommendations solution has helped to increase year-over-year time-on-page by 10 percent and pageviews by 9.42 percent. It has also decreased searches without results by 30 percent in two short months.
Get ready to be inspired and learn more about Mathematica’s success story.