If you still have questions about the term metadata, you’re not alone. Many data management professionals have spent countless hours studying metadata. I’m one of them, and in this article, I’ll do my best to answer the question, What is metadata? while providing some advice on how to use and manage it effectively as a critical component of your data governance strategy.
The word metadata roughly translates as “data beyond data,” given that meta (Greek μετα) means “past” or “beyond.” You’ve probably heard of a meta tag like a meta title or meta description. Definitely different permutations, but I’d like to cover the topic with more depth.
As for a definition, let’s use this for the purposes of this article:
What is Metadata?
Metadata is data about data that can be used to describe, categorize, and manage digital assets. Broadly speaking, search engines use metadata to accurately understand, index, and rank content in search results, with the goal of making each search result as relevant as possible. Metadata plays an integral role in data quality management, data lineage tracking, and ensuring proper data access across organizations.
Simple enough. But before we dig into its complexities and value to search engines, you need to know what data is and how metadata transforms a piece of raw information into a valuable, discoverable data asset.
How Metadata Came to Be
One of the earliest and most tangible examples of metadata dates back to 280 BC, when the Great Library of Alexandria attached small tags to the end of scrolls. The tags contained important (scannable) information for library users: title, subject, and author. They made it easy to identify a scroll’s content without unrolling each scroll.
The tags also helped librarians return materials to their proper locations. This early metadata system eventually evolved into library card catalogs, which served as comprehensive metadata repositories for centuries.
Relevant reading: History of Information Retrieval
Now let’s fast forward to the earliest days of business computing. Back then, most computer usage involved recording transactions, like an order or invoice, and then fulfilling that order. While there were a few data models at the time, people typically stored the data tape, using magnetic sections to store binary information in fixed-length chunks called records.
If you wanted to store information about where someone lived in order to send a bill, then all of the fields about the address had to be kept in the same record.
Eventually, people came up with the idea of joining rows of two tables together by using special markers called keys, where one key (the primary key) identified a row in a table, while another key (the foreign key) stored this same number as a pointer in a different field of another table.
Using records and keys, you could create a table of bills and a table of addresses, instead of each record having to store the same information over and over again. Then the bill record could just point to the address record, significantly reducing the amount of data needed to be captured — and the row in the referenced address table could then be said to be about the address being referenced.
Here you have some of the earliest examples of metadata management.
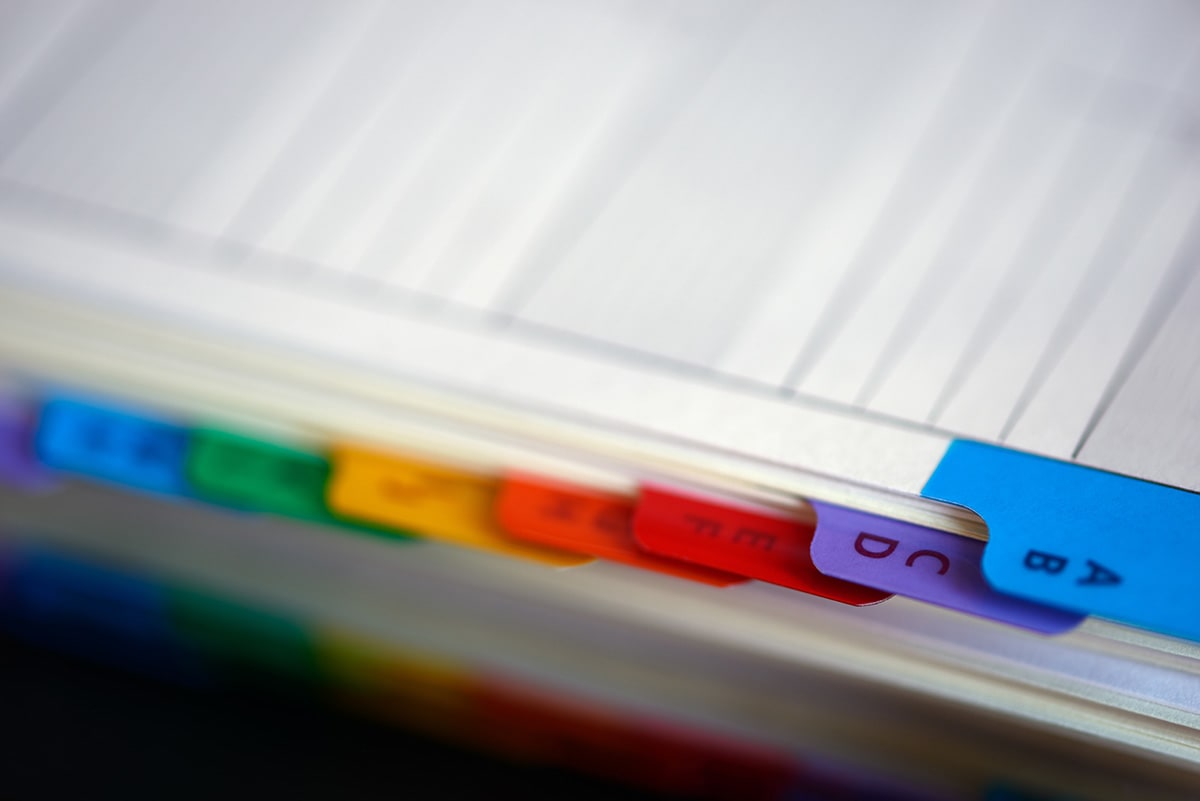
In other words, the row that contained the address could be considered metadata about the address reference in the bill listing.
The implication of this is important: metadata is simply data that describes (is about) other data. Sometimes that metadata was made explicit in the data model, such as in the bill and address example given above. Other times, the metadata is more subtle and implicit, representing assumed information that may or may not be captured in the model, e.g., tags. This implicit information often becomes critical for effective data discovery and data access.
In enterprise search, metadata is useful for organizing, grouping, and navigating all of the documents and content resources amassed by an enterprise throughout its existence. An enterprise search engine uses metadata, among other things, to create a relevant search result page for the searcher. Good metadata enhances data quality and provides relevant information that improves search results.
However, barriers can arise when different index owners or taxonomy managers use different terms to describe what are essentially the same digital assets—and this is where artificial intelligence can be helpful.
The Different Types of Metadata
Different kinds of metadata include, but certainly aren’t limited to:
Descriptive metadata
Essential for effective data discovery, descriptive metadata helps transform raw information into searchable digital assets. It includes basic information like category, topic, author, type of asset like a white paper, video, or web page (tag, abstract, creation date, and so on), and even physical products. This is ideal for allowing knowledge articles to be classified.
For example, when you search for a book on Amazon, the descriptive metadata includes the book title, author name, publication date, and genre. In a corporate setting, a document’s descriptive metadata could include the document title, creator, department, and subject matter.
Product metadata
Used to manage data in ecommerce environments, product metadata lists the attributions and descriptions of a product. This type of metadata creates structured data that enhances searchability and user experience. Metadata can include a style, brand name, occasions in which something might be used, color, size, is it sustainable — all these descriptors can then be exposed as faceted navigation for the shopper.
When browsing a fashion brand’s website for a new dress, product metadata lets the shopper filter results by size, color, price range, and material. In a B2B context, product metadata might include specifications, compatibility information, warranty details, and inventory status.
Structural metadata
Structural metadata is critical for effective data governance and management. It involves the structure of database objects like indexes, tables, columns, or keys. This type of metadata serves as the architectural blueprint for how data assets are organized within a system, enabling efficient data access and retrieval.
For example, in a relational database, structural metadata defines how tables are connected through primary and foreign keys, specifying relationships between customer records and their purchase history. In content management systems, structural metadata might define how pages are organized hierarchically, including parent-child relationships between different sections of a website or digital resource.
Administrative metadata
A foundational element of data governance frameworks, this metadata type supports data security, access control, and lifecycle management of digital resources. Administrative metadata acts as a guide, helping humans navigate data assets. It includes information on managing a resource; preservation metadata falls under this category, meaning information on how to save a resource as does the date an asset was created or modified.
For example, in a document management system, administrative metadata tracks who created a file, who has permission to view or edit it, when it was last modified, and its retention schedule. For digital collections in libraries or archives, administrative metadata might include copyright information, usage restrictions, and preservation requirements — all critical metadata records that ensure proper digital asset management and stewardship of information resources.
Technical metadata
Technical data is focused on maintaining data quality and interoperability. It’s another category of guide metadata that describes the structure of information in a data warehouse or business intelligence system. This metadata type documents the technical characteristics of data sets and elements, supporting data discovery and integration across systems.
For example, in a media asset management system, technical metadata might include file format, resolution, compression method, and encoding specifications for videos or images. In a data catalog, technical metadata could specify data types, field lengths, validation rules, and transformation logic — providing critical context for data scientists and analysts working with these resources.
Schematic metadata
A schema describes the allowable relationships that a metadata element can have with other elements in the data system, along with constraints on those relationships. In the case of relational databases, the schema structure is defined explicitly ahead of time, because the database needs this information to tell it how to both interpret and efficiently store certain types of data (such as numbers or dates).
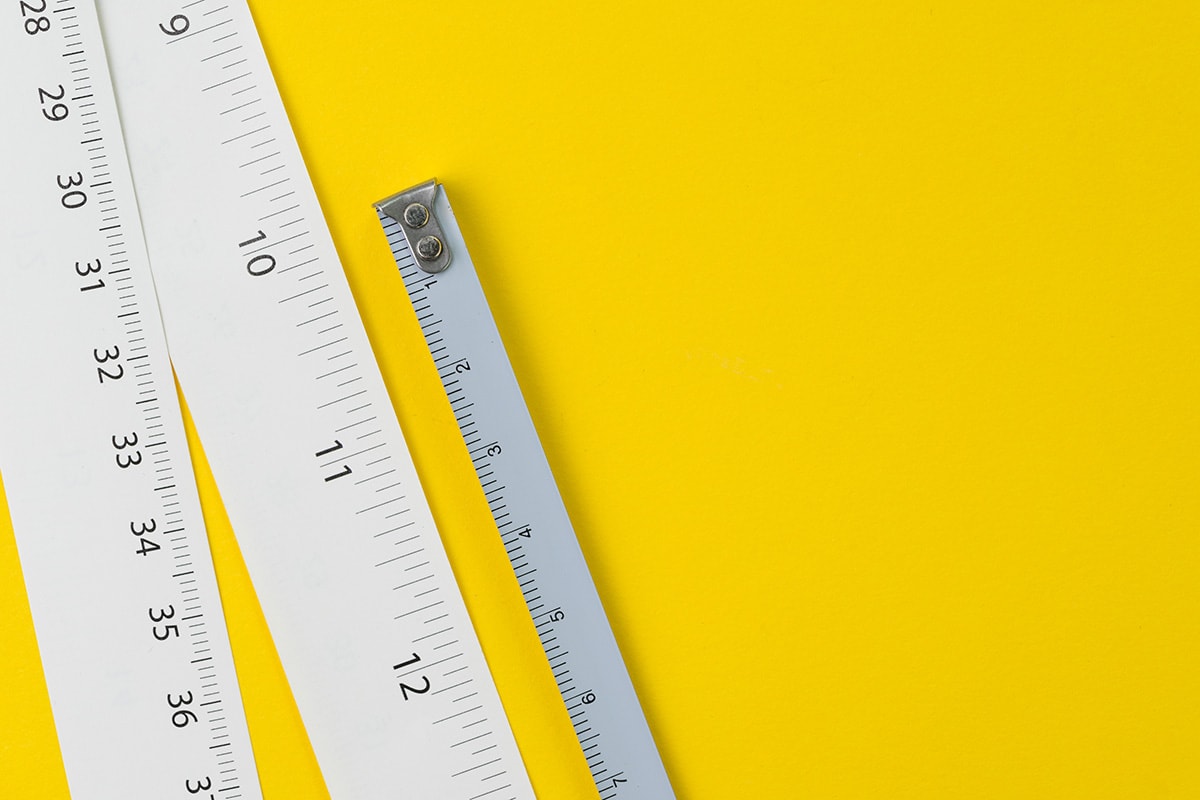
Content schemas (or document metadata), such as those used to describe a webpage, Microsoft Word documents, or the puppy and kitten videos you watch during your lunch break, are usually implicit and externally applied. This means that the system can interpret the text even without the schema but can accept or reject the validity of that document (a process called schema validation) based upon the schema in a more advisory approach. Schema-less systems still have an implicit structure, it’s just that the structure is not necessarily available to the machine to use.
Reifications and Annotations
Finally, in some data storage representations such as graphs, where you can create assertions (observed facts), you can create a form of metadata known as a reification. Reifications are part of a broader class of metadata called annotations. An annotation is a comment about another statement or set of statements, and may provide additional classification, descriptions, alternative phrases, and provenance metadata (where the statement was made and who reported it).
When you see activity streams on social media, for example, you’re looking at annotations about statements being made. And as an example of a reification, suppose that you have the following statement (an assertion):
“Jane spent $45 for a blouse.”
If you wanted to provide some kind of context about the statement, such as:
“Mark reported that Jane spent $45 for a blouse.”
This would be considered a reification — a statement about a statement.
Metadata and Classification
Metadata plays an important role in classification systems, which are used to improve workflow efficiency, reduce errors, and make information easier to find.
In practice, database developers implement classification by creating specific fields in tables (like an address_type table) that store metadata about different categories. They then create user interface elements, such as dropdown menus, allowing users to select the appropriate category. This structured approach to classification significantly improves user experience and data organization.
For example, in a corporate document management system, documents are classified using metadata tags for department (HR, Finance, Marketing), document type (policy, report, presentation), confidentiality level (public, internal, confidential), and status (draft, under review, approved). When a user uploads a new policy document, they assign these classification tags through a form interface. Later, these classifications enable powerful filtering capabilities — allowing users to quickly find, for example, “all approved HR policies” or “all confidential financial reports under review.”
Metadata classification, when implemented correctly, transforms raw information into structured data that becomes a valuable data asset for our organization. The metadata doesn’t just describe the documents, but creates relationships between them and establishes a framework for how they should be processed, accessed, and maintained.
Dimensional Analysis as Metadata
Dimensional analysis is a process of decomposing a complex problem into smaller, more manageable dimensions. These dimensions can then be used to analyze the problem and to identify solutions.
Consider a situation where an architect stores information about materials used in the construction of a house. The type of wood beams, for example, or the size of the chimney, as numbers in metadata fields with names like fireplace length, fireplace_height and so forth, with their lengths given as 8.25, 23.75, 2.5, etc.
Now, let’s break this down step by step:
1. The architect records measurements (8.25, 23.75, 2.5) in the database
2. These numbers represent dimensions of a fireplace
3. Without proper metadata, these numbers lack context
If you happen to be familiar with fireplaces or construction and had grown up in the United States, you would assume that these numbers were in units of feet. If, however, you had learned about architecture in Europe, your first assumption would likely be that these dimensions were given in meters. This could make for a huge fireplace.
A computer, of course, would have no clue about what the unit of measurement was, and if not defined, a 3D printer might very well give you outputs perfect for a giant fireplace, when what you really wanted was… a normal one.
This is why units are essential metadata — they provide context for interpreting numerical values. Without this metadata, the same numbers could lead to dramatically different outcomes.
Metadata are critical pieces of information about the interpretation of content fields that aren’t necessarily stored with that content. This can be especially important with unstructured data, also referred to as unstructured content.
In a relational database, a (bad) solution would be to add a unit description into the metadata field name. This would tell the database user how to interpret the units — but doesn’t necessarily tell the computer itself without parsing out the name. A better solution would be to set up a table that consisted of property names per table and that identified the dimension of a given field based upon the metadata for that field.
How to Optimize Metadata for Your Search Engine
Metadata plays a critical role in the end-user experience because it connects searchers to relevant information. What your ecommerce buyer sees on a product results page, the text snippets included in site search engine results — it’s all influenced by metadata.
As such, adhere to a few best practices to ensure your metadata is optimized for your search engine:
- Identify the most important metadata fields for your search index.
- Map metadata fields to the appropriate data types.
- Normalize metadata values to ensure consistency.
- Use metadata to create facets and filters for better navigation.
- Prioritize certain content in search results by assigning higher relevance scores to metadata fields.
- Use metadata to manage unstructured content by concatenating fields or adding them to the document body.
- Personalize search results using metadata like user location or purchase history.
- Regularly update and maintain metadata to keep it accurate and relevant.
- Have a system in place for handling special cases and exceptions.
The importance of mapping metadata to the appropriate fields in a search index shouldn’t be understated. Check out Mapping Metadata: Tips for Best Search Results for more on how to map metadata fields with Coveo.
Maintaining Metadata
One last important point to consider: metadata typically provides context to data. It’s the information that the data itself is implicitly assuming in order to be true or meaningful. Because context often falls outside of the structure of that data , you should be careful about working with any system or metadata management tool that bills itself as a “metadata server.”
They can determine the potential context of information based on what’s known about existing information, but you should make sure you have a way to validate that the contextual metadata so produced is in fact correct and consistent.
And that’s another issue. Metadata can become stale. Maintaining without the help of AI automation is challenging (if not impossible) due to inconsistencies, outdated values, and the sheer volume of data across systems. Poor metadata quality can lead to irrelevant search results and a degraded user experience. This makes regular data cleaning essential for accuracy consistency. Strategies to address this include:
- Leveraging a unified index that normalizes metadata values
- Automating data management with AI
- Using abilities to identify and resolve errors
To explore data cleaning best practices and strategies, download our ebook, the Best Data Cleaning Practices for Search Experiences.
When you are talking about enterprise-sized repositories, maintaining metadata at scale is hard. However, good machine learning can eliminate some of the need for maintenance as it learns from users’ behaviors.
Summary
There is no question that metadata is important in computing, especially as vector search, machine learning, and natural language processing all become central to metadata and management overall. As big data grows and new AI capabilities like generative AI and agentic AI become increasingly integrated with site search, focusing on metadata management will ensure your company information is optimized for search and can deliver accurate AI-driven insights. Hopefully, this brief overview provides a good reference for your own work with metadata.
Dig Deeper
Your enterprise’s metadata standard will vary depending on a number of factors, and taxonomy is one of them. But there are some myths floating around about the importance and prioritization of taxonomy when it comes to enterprise search. Learn more about the three myths of data taxonomy (a scheme of classification) slowing down your digital transformation.